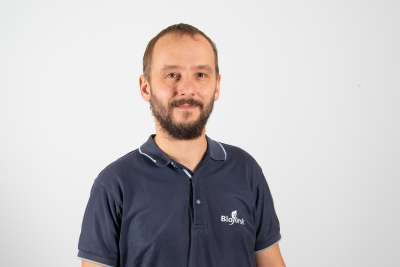
Senter for presisjonsjordbruk
Senter for presisjonsjordbruk ligger på NIBIO Apelsvoll ved Kapp i Østre Toten. Senteret ble etablert i 2016. Senterets formål er å bidra til et ressurseffektivt og bærekraftig jordbruk gjennom å gjøre vegen kortere fra ny teknologi utvikles til den kommer bonden til gode.
Litt om presisjonsjordbruk
Hovedmålet med presisjonsjordbruk (PJ) er å optimere avlinger og kvalitet samtidig som en tar vare på naturmiljøet, slik at en oppnår både økonomiske og miljømessige fordeler.
Presisjonsjordbruk er en produksjonsstrategi som bygger på innsamling, bearbeiding og analyse av temporale, romlige og individspesifikke data. Denne informasjonen kombineres med annen kunnskap om produksjonssystemet for å styre tiltak etter den identifiserte variasjonen og oppnå bedre ressursutnyttelse, produktivitet, kvalitet, lønnsomhet og bærekraft. Presisjonsjordbruk handler altså om å bruke ny teknologi til å tilpasse behandlingen av jord og vekst etter behovet, som ofte varierer mye innenfor samme jordet.
Tradisjonelt behandles hele jordet likt ut fra det en mener er riktig i gjennomsnitt. Ved presisjonsjordbruk tilpasses for eksempel gjødselmengden, sprøytemengden og kalkmengden ut fra det stedsspesifikke behovet. Dette behovet kartlegges ved å sette sammen informasjon fra mange kilder, der ulike sensorer, kamera og globale navigasjonssystemer (GNSS) spiller en sentral rolle. Slikt utstyr kan monteres på for eksempel traktorer, roboter, droner eller satellitter.
Hva jobber vi med på senteret?
Vårt team av forskere og ingeniører ved Senter for presisjonsjordbruk arbeider med å utvikle ny teknologi og metodikk knyttet til fjernanalyse av planteegenskaper, automatisering av ulike agronomiske prosesser og formidling av data og metoder.
Vi forsker på vekster som korn, bær, eng og poteter og utvikler metoder for å ekstrahere informasjon om plantenes nitrogeninnhold, vannstatus og helsesituasjon. Videre utvikler vi modeller for å prognostisere avlinger og produktkvalitet relativt tidlig i vekstsesongen.
Vi utvikler og tester teknologiske løsninger både for dagens og framtidas presisjonsjordbruk. Vår FoU-aktivitet er konsentrert rundt temaene:
- Presisjonsgjødsling
- Presisjonsplantevern
- Fjernmåling og spektroskopi
- Digitale tjenester for smart landbruk
- Maskinlæring og kunstig intelligens
- Landbruksteknikk og automatisering
Vi samarbeider med både nasjonale og internasjonale aktører som sammen dekker et vidt spenn – fra sluttbrukere via sentrale markedsaktører i landbruket til teknologibedrifter. I tillegg har vi et utstrakt samarbeid med forskningsmiljøer over hele verden.
FoU-temaer
Publikasjoner
Sammendrag
Introduction: Production of strawberries in greenhouses and polytunnels is gaining popularity worldwide. This study investigated the effect of reuse of coir and peat, two substrates commonly adapted to soilless strawberry production, as well as stand-alone wood fiber from Norway spruce, a promising substrate candidate. Methods: The experiment was performed in a polytunnel at NIBIO Apelsvoll, Norway, and evaluated both virgin substrates, as well as spent materials that were used in one or two years. Yield, berry quality and plant architecture of the strawberry cultivar ‘Malling Centenary’ were registered. In addition, chemical and physical properties of virgin and reused substrates were investigated. Results: While plants grown in peat and wood fiber had highest yield in the first year of production, the berry yield was slightly reduced when these substrates were utilized for the second and third time. However, yield was comparable to the yield level attained in new and reused coir. Interestingly, berries grown in wood fiber had a tendency to a higher sugar accumulation. This substrate also produced the highest plants. Stand-alone wood fiber was the substrate with the highest accumulation of nitrogen during the three consecutive production cycles. All three investigated materials revealed a trend for decreased potassium accumulation. Wood fiber is characterized by the highest percentage of cellulose, however after three years of production the cellulose content was reducedto the same levels as for coir and peat. Discussion: Implementation of wood fiber as a growing medium, as well as general practice of substrate reuse can be therefore an achievable strategy for more sustainable berry production.
Sammendrag
Wood modification using polyesterification of sorbitol and citric acid is a novel environmentally friendly strategy for wood protection improving its dimensional stability and acts against fungal deterioration. Inelastic Raman scattering is sensitive to the molecules of high polarizability and both lignocellulose and aliphatic esters formed during the treatment are polar. Therefore, in the present study, the quality control of the treatment using a handheld Raman spectrometer equipped with 830 nm laser is suggested as a rapid and reliable approach. Raman spectra from six wood modification levels (resulting in different weight percent gain, WPG) of three different wood species (Silver birch, Scots pine and Norway spruce) as well as three sample preparation strategies (intact, sanded and milled wood samples) were collected, and further analyzed using a chemometric method. Best performing models based on Powered Partial Least Squares Regression predicted the WPG level at R2 = 0.85, 0.95 and 0.98 for birch, pine and spruce, respectively. In addition, a clear separation between hard and soft wood species was also captured. Especially for softwood species, the sample preparation method affected the model accuracy, revealing the best performance in milled material. It is concluded that by using handheld Raman spectrometer it is possible to perform accurate quality control of wood modified by polyesterification of citric acid and sorbitol.
Sammendrag
The ageing population, climate change, and labour shortages in the agricultural sector are driving the need to reevaluate current farming practices. To address these challenges, the deployment of robot systems can help reduce environmental footprints and increase productivity. However, convincing farmers to adopt new technologies poses difficulties, considering economic viability and ease of use. In this paper, we introduce a management system based on the Robot Operating System (ROS) that integrates heterogeneous vehicles (conventional tractors and mobile robots). The goal of the proposed work is to ease the adoption of mobile robots in an agricultural context by providing to the farmer the initial tools needed to include them alongside the conventional machinery. We provide a comprehensive overview of the system’s architecture, the control laws implemented for fleet navigation within the field, the development of a user-friendly Graphical User Interface, and the charging infrastructure for the deployed vehicles. Additionally, field tests are conducted to demonstrate the effectiveness of the proposed framework.
Sammendrag
A process-based model was developed to predict dry matter yields and amounts of harvested nitrogen in conventionally cropped grassland fields, accounting for within-field variation by a node network design and utilizing remotely sensed information from a drone-borne system for increased accuracy. The model, named NORNE, was kept as simple as possible regarding required input variables, but with sufficient complexity to handle central processes and minimize prediction errors. The inputs comprised weather data, soil information, management data related to fertilization, and a visual estimate of clover proportion in the aboveground biomass. A sensitivity analysis was included to apportioning variation in dry matter yield outputs to variation in model parameter settings. Using default parameter values from the literature, the model was evaluated on data from a two-year study (2016–2017, 264 research plots in total each year) conducted at two locations in Norway (i.e. in South-East and in Central Norway) with contrasting climatic conditions and with internal variation in soil characteristics. The results showed that the model could estimate dry matter yields with a relatively high accuracy without any corrections based on remote sensing, compared with published results from comparable model studies. To further improve the results, the model was calibrated shortly before harvest, using predictions of above ground dry matter biomass obtained from a drone-borne remote sensing system. The only parameters which were hereby adjusted in the NORNE model were the starting values of nitrogen content in soil (first cut) and the plant available water capacity (second cut). The calibration based on the remotely sensed information improved the predictive performance of the model significantly. At first cut, the root mean square error (RMSE) of dry matter yield prediction was reduced by 20% to a mean value of 58 g m−2, corresponding to a relative value (rRMSE) of 0.12. For the second cut, the RMSE decreased by 13% to 66 g m−2 (rRMSE: 0.18). The model was also evaluated in terms of the predictions of amounts of nitrogen in the harvested crop. Here, the calibration reduced the RMSE of the first cut by 38%, obtaining a mean RMSE value of 2.1 g N m−2 (rRMSE: 0.28). For the second cut, the RMSE reduction for simulated harvested N was 16%, corresponding to a mean RMSE value of 2.3 g N m−2 (rRMSE: 0.33). The large improvements in model accuracy for simulated dry matter and nitrogen yields obtained through calibration by utilizing remotely sensed information, indicate the importance of considering spatial variability when applying models under Nordic conditions, both for yield predictions and for decision support for nitrogen application.
Sammendrag
Weeds affect crop yield and quality due to competition for resources. In order to reduce the risk of yield losses due to weeds, herbicides or non-chemical measures are applied. Weeds, especially creeping perennial species, are generally distributed in patches within arable fields. Hence, instead of applying control measures uniformly, precision weeding or site-specific weed management (SSWM) is highly recommended. Unmanned aerial vehicle (UAV) imaging is known for wide area coverage and flexible operation frequency, making it a potential solution to generate weed maps at a reasonable cost. Efficient weed mapping algorithms need to be developed together with UAV imagery to facilitate SSWM. Different machine learning (ML) approaches have been developed for image-based weed mapping, either classical ML models or the more up-to-date deep learning (DL) models taking full advantage of parallel computation on a GPU (graphics processing unit). Attention-based transformer DL models, which have seen a recent boom, are expected to overtake classical convolutional neural network (CNN) DL models. This inspired us to develop a transformer DL model for segmenting weeds, cereal crops, and ‘other’ in low-resolution RGB UAV imagery (about 33 mm ground sampling distance, g.s.d.) captured after the cereal crop had turned yellow. Images were acquired during three years in 15 fields with three cereal species (Triticum aestivum, Hordeum vulgare, and Avena sativa) and various weed flora dominated by creeping perennials (mainly Cirsium arvense and Elymus repens). The performance of our transformer model, 1Dtransformer, was evaluated through comparison with a classical DL model, 1DCNN, and two classical ML methods, i.e., random forest (RF) and k-nearest neighbor (KNN). The transformer model showed the best performance with an overall accuracy of 98.694% on pixels set aside for validation. It also agreed best and relatively well with ground reference data on total weed coverage, R2 = 0.598. In this study, we showed the outstanding performance and robustness of a 1Dtransformer model for weed mapping based on UAV imagery for the first time. The model can be used to obtain weed maps in cereals fields known to be infested by perennial weeds. These maps can be used as basis for the generation of prescription maps for SSWM, either pre-harvest, post-harvest, or in the next crop, by applying herbicides or non-chemical measures.
Forfattere
Jiangsan ZhaoSammendrag
Det er ikke registrert sammendrag
Forfattere
El Houssein Chouaib HarikSammendrag
In this paper, we investigated the idea of including mobile robots as complementary machinery to tractors in an agricultural context. The main idea is not to replace the human farmer, but to augment his/her capabilities by deploying mobile robots as assistants in field operations. The scheme is based on a leader–follower approach. The manned tractor is used as a leader, which will be taken as a reference point for a follower. The follower then takes the position of the leader as a target, and follows it in an autonomous manner. This will allow the farmer to multiply the working width by the number of mobile robots deployed during field operations. In this paper, we present a detailed description of the system, the theoretical aspect that allows the robot to autonomously follow the tractor, in addition to the different experimental steps that allowed us to test the system in the field to assess the robustness of the proposed scheme.
Forfattere
Siv Mari Aurdal Tomasz Leszek Woznicki Trond Haraldsen Krzysztof Kusnierek Anita Sønsteby Siv Fagertun RembergSammendrag
Cultivation of strawberries in greenhouses and polytunnels is increasing, and new sustainable growing media are needed to replace peat and coconut coir. This study investigated the effect of wood fiber and compost as growing media on hydroponically cultivated strawberries. Two experiments were conducted, where the everbearing cultivar ‘Murano’ was grown in mixtures of wood fiber and compost (Experiment 1) and the seasonal flowering cultivar ‘Malling Centenary’ was grown in mixtures of wood fiber and peat (Experiment 2). Additionally, in Experiment 2, the effect of adding start fertilizer was assessed. The yield potential of ‘Murano’ plants was maintained in all substrates compared to the coconut coir control. However, a mixture of 75% wood fiber and 25% compost produced the highest yield, suggesting that mixtures of nutritious materials with wood fiber may improve plant performance. The chemical composition of the berries was not affected by the substrate composition; however, berries from plants grown in the best performing blend had a lower firmness than those grown in coconut coir. ‘Malling Centenary’ plants produced higher yields in substrates enriched with start fertilizer. Generally, the productivity of ‘Malling Centenary’ plants was maintained in blends containing up to 75% of wood fiber mixture even without start fertilizer.
Forfattere
Jiangsan ZhaoSammendrag
Det er ikke registrert sammendrag
Forfattere
Jiangsan ZhaoSammendrag
Det er ikke registrert sammendrag
Sammendrag
Rapid methods allowing for non-destructive crop monitoring are imperative for accurate in-season nitrogen (N) status assessment and precision N management. The objectives of this paper were to (1) compare the performance of a leaf fluorescence sensor Dualex 4 and an active canopy reflectance sensor Crop Circle ACS-430 for estimating maize (Zea mays L.) N status indicators across growth stages; (2) evaluate the potential of N status prediction across growth stages using the reflectance parameters acquired from the canopy sensor at an early growth stage; and, (3) investigate the prospect of combining the active canopy sensor and leaf fluorescence sensor data to estimate N nutrition index (NNI) indirectly using a general model across growth stages. The results indicated that data from both sensors were closely related to NNI across stages. However, using the direct NNI estimation method, among the tested indices, only the N balance index (NBI) could diagnose N status satisfactorily, based on the Kappa statistics. The effect of growth stages on proximal sensing was reduced by incorporating the information of days after sowing. It was found that the leaf fluorescence sensor performed relatively better in estimating plant N concentration whereas the canopy reflectance sensor performed better in aboveground biomass estimation. Their combination significantly improved the reliability of N diagnosis, including NNI prediction. In addition, the study confirmed that N status can be assessed by predicting aboveground biomass at the later stages using the canopy reflectance measurements at an early stage. Furthermore, the integrated NBI was verified to be a more robust and sensitive N status indicator than the chlorophyll concentration index. It is concluded that combining active canopy sensor data, of an early growth stage (e.g. V8), with leaf fluorescence sensor data, modified using days after sowing, can improve the accuracy of corn N status diagnosis across growth stages.
Sammendrag
Active crop sensor-based precision nitrogen (N) management can significantly improve N use efficiency but generally does not increase crop yield. The objective of this research was to develop and evaluate an active canopy sensor-based precision rice management system in terms of grain yield and quality, N use efficiency, and lodging resistance as compared with farmer practice, regional optimum rice management system recommended by the extension service, and a chlorophyll meter-based precision rice management system. Two field experiments were conducted from 2011 to 2013 at Jiansanjiang Experiment Station of China Agricultural University in Heilongjiang, China, involving four rice management systems and two varieties (Kongyu 131 and Longjing 21). The results indicated that the canopy sensor-based precision rice management system significantly increased rice grain yield (by 9.4–13.5%) over the farmer practice while improving N use efficiency, grain quality, and lodging resistance. Compared with the already optimized regional optimum rice management system, in the cool weather year of 2011, the developed system decreased the N rate applied in Kongyu 131 by 12% and improved N use efficiency without inducing yield loss. In the warm weather year of 2013, the canopy sensor-based management system recommended an 8% higher N rate to be applied in Longjing 21 than the regional optimum rice management, which improved rice panicle number per unit area and eventually led to increased grain yield by over 10% and improved N use efficiency. More studies are needed to further test the developed active canopy sensor-based precision rice management system under more diverse on-farm conditions and further improve it using unmanned aerial vehicle or satellite remote sensing technologies for large-scale applications.
Forfattere
Erika Krüger Tomasz Leszek Woznicki Ola M. Heide Krzysztof Kusnierek Rodmar Isak Rivero Agnieszka Masny Iwona Sowik Bastienne Brauksiepe Klaus Eimert Daniela Mott Gianluca Savini Marino Demene Karine Guy Aurelie Petit Béatrice Denoyes Anita SønstebySammendrag
Det er ikke registrert sammendrag
Sammendrag
The remote sensing of the biophysical and biochemical parameters of crops facilitates the preparation of application maps for variable-rate nitrogen fertilization. According to comparative studies of machine learning algorithms, Gaussian process regression (GPR) can outperform more popular methods in the prediction of crop status from hyperspectral data. The present study evaluates GPR model accuracy in the context of spring wheat dry matter, nitrogen content, and nitrogen uptake estimation. Models with the squared exponential covariance function were trained on images from two hyperspectral cameras (a frenchFabry–Pérot interferometer camera and a push-broom scanner). The most accurate predictions were obtained for nitrogen uptake (R2=0.75–0.85, RPDP=2.0–2.6). Modifications of the basic workflow were then evaluated: the removal of soil pixels from the images prior to the training, data fusion with apparent soil electrical conductivity measurements, and replacing the Euclidean distance in the GPR covariance function with the spectral angle distance. Of these, the data fusion improved the performance while predicting nitrogen uptake and nitrogen content. The estimation accuracy of the latter parameter varied considerably across the two hyperspectral cameras. Satisfactory nitrogen content predictions (R2>0.8, RPDP>2.4) were obtained only in the data-fusion scenario, and only with a high spectral resolution push-broom device capable of capturing longer wavelengths, up to 1000 nm, while the full-frame camera spectral limit was 790 nm. The prediction performance and uncertainty metrics indicated the suitability of the models for precision agriculture applications. Moreover, the spatial patterns that emerged in the generated crop parameter maps accurately reflected the fertilization levels applied across the experimental area as well as the background variation of the abiotic growth conditions, further corroborating this conclusion.
Sammendrag
Accurate and non-destructive diagnosis of crop nitrogen (N) surplus and deficit status based on N nutrition index (NNI) is crucially important for the success of precision N management to improve N use efficiency (NUE) and reduce negative environmental impacts. However, due to the variability of the reflectance data obtained from different active crop sensors and complexity of the environmental and management conditions for regional applications, accurate determination of crop N status and topdressing N rate only using active canopy sensor data is very challenging. The objectives of this study were to (1) develop an in-season N status diagnosis and recommendation model based on NNI prediction using multi-source data fusion with machine learning, and (2) evaluate the accuracy of N diagnosis and recommendation in terms of rice yield and NUE under diverse on-farm conditions. Thirty plot experiments and thirteen on-farm experiments were conducted in Qixing Farm, Jiansanjiang, Northeast China from 2008 to 2018, and the dataset was used for the model calibration, validation, and evaluation. Two indirect and one direct NNI prediction methods using simple regression, stepwise multiple linear regression (SMLR) and random forest regression (RFR) were compared for N diagnosis and then integrated into N recommendation model. The results indicated that combining environmental and agronomic variables with crop sensor data improved the SMLR and RFR model performance by 1–16% and 9–40% over the corresponding models only using crop sensor data, respectively. The direct NNI prediction approach achieved slightly better N status diagnostic accuracy (areal agreement = 84% and Kappa statistics = 0.71) than indirect NNI prediction strategies based on plant N uptake and ΔN estimation (areal agreement = 81% and Kappa statistics = 0.67) or aboveground biomass and plant N uptake estimation (areal agreement = 77% and Kappa statistics = 0.58) across plot experiments and diverse on-farm conditions, based on multi-source data fusion with random forest regression models. About 82% of recommended N rates by the developed integrated in-season rice N diagnosis and recommendation model were within ±10 kg ha−1 of the measured economic optimum N rate across different varieties, environmental conditions and transplanting densities. Precision rice management based on in-season N diagnosis and recommendation decreased N rates and increased N partial factor productivity (PFPN) by 23% over regional optimum rice management, and significantly increased yield (7–11%) and PFPN (33–77%) over farmer's management. More studies are needed to develop in-season N diagnosis and recommendation strategies for applications across different regions and combine them with integrated precision rice management strategies for food security and sustainable development.
Sammendrag
Det er ikke registrert sammendrag
Sammendrag
Horticultural production systems are under pressure to find environmentally friendly growing media. Peat is currently the most popular substrate for fresh potted herbs production; however, this raw material is not sustainable due to the large amount of greenhouse gases released during its harvesting. Therefore, the goal of the study was to test the performance of various commercial wood fiber products and compare them with peat and coir in an ebb-and-flow production system with basil (Ocimum basilicum L. 'Marian'). Basil plants were grown in three different pot sizes (6, 9 and 12 cm in diameter) and under various fertigation regimes (EC 1, 2 and 3). Height and biomass of the plants were recorded when the best performing plants reached the commercial stage. The tallest plants and greatest biomass were produced in peat and coir, however, the results confirm that wood fiber can be a promising substrate alternative. Further research is needed to study, among others topics, how to modify some properties of wood fibers to fulfil their potential as a replacement for non-sustainable growing media in production of herbs in pots.
Sammendrag
Accurate assessment of crop nitrogen (N) status and understanding the N demand are considered essential in precision N management. Chlorophyll fluorescence is unsusceptible to confounding signals from underlying bare soil and is closely related to plant photosynthetic activity. Therefore, fluorescence sensing is considered a promising technology for monitoring crop N status, even at an early growth stage. The objectives of this study were to evaluate the potential of using Multiplex® 3, a proximal canopy fluorescence sensor, to detect N status variability and to quantitatively estimate N status indicators at four key growth stages of maize. The sensor measurements were performed at different growth stages, and three different regression methods were compared to estimate plant N concentration (PNC), plant N uptake (PNU), and N nutrition index (NNI). The results indicated that the induced differences in maize plant N status were detectable as early as the V6 growth stage. The first method based on simple regression (SR) and the Multiplex sensor indices normalized by growing degree days (GDD) or N sufficiency index (NSI) achieved acceptable estimation accuracy (R2 = 0.73–0.87), showing a good potential of canopy fluorescence sensing for N status estimation. The second method using multiple linear regression (MLR), fluorescence indices and GDDs had the lowest modeling accuracy (R2 = 0.46–0.79). The third tested method used a non-linear regression approach in the form of random forest regression (RFR) based on multiple sensor indices and GDDs. This approach achieved the best estimation accuracy (R2 = 0.84–0.93) and the most accurate diagnostic result.
Sammendrag
One challenge in precision nitrogen (N) management is the uncertainty in future weather conditions at the time of decision-making. Crop growth models require a full season of weather data to run yield simulation, and the unknown weather data may be forecasted or substituted by historical data. The objectives of this study were to (1) develop a model-based in-season N recommendation strategy for maize (Zea mays L.) using weather data fusion; and (2) evaluate this strategy in comparison with farmers’ N rate and regional optimal N rate in Northeast China. The CERES-Maize model was calibrated using data collected from field experiments conducted in 2015 and 2016, and validated using data from 2017. At two N decision dates - planting stage and V8 stage, the calibrated CERES-Maize model was used to predict grain yield and plant N uptake by fusing current and historical weather data. Using this approach, the model simulated grain yield and plant N uptake well (R2 = 0.85–0.89). Then, in-season economic optimal N rate (EONR) was determined according to responses of simulated marginal return (based on predicted grain yield) to N rate at planting and V8 stages. About 83% of predicted EONR fell within 20% of measured values. Applying the model-based in-season EONR had the potential to increase marginal return by 120–183 $ ha−1 and 0–83 $ ha−1 and N use efficiency by 8–71% and 1–38% without affecting grain yield over farmers’ N rate and regional optimal N rate, respectively. It is concluded that the CERES-Maize model is a valuable tool for simulating yield responses to N under different planting densities, soil types and weather conditions. The model-based in-season N recommendation strategy with weather data fusion can improve maize N use efficiency compared with current farmer practice and regional optimal management practice.
Forfattere
Tomasz Leszek Woznicki Krzysztof Kusnierek Unni Myrheim Roos Sofie Andersen Katrin Zimmer Anita SønstebySammendrag
The use of peat as a growing media in horticulture is supposed to be reduced due to negative effects of its production on the environment. Interest in development of alternative growing media is therefore increasing and is enhanced by both political pressure and industry demands. Therefore, the influence of 33 growing media on the performance and productivity of two strawberry cultivars were examined in a polytunnel under Nordic conditions (60.7 N). Alternative substrates including fibers of spruce, birch and flax and coffee grounds were tested standalone or in mixes. Peat and coir were included as controls. Additionally, impregnation of the wood fibers with organic and inorganic substances was examined. All investigated growing media received identical fertigation strategies (EC 1.5). The highest average biomass production was observed for plants grown in bare peat; however, the best yield performance was noted for peat mixed with perlite and for coarse spruce fiber. Strawberries grown in these two best performing substrates showed comparable overall productivity, with 272 and 268 g of berries per plant, respectively. Both peat/perlite mix and the coarse spruce fiber had also a similar weight of berries larger than 25 mm, with 210 and 198 g plant-1, respectively. Moreover, improvement of the substrate structure by adding perlite or wood chips may have had a pronounced effect on fruiting performance. When compared to peat with added perlite (which gave the highest berry yield in the experiment; 272 g plant-1), strawberries grown in pure peat produced only 187 g plant-1. Furthermore, impregnation of spruce fiber with humic acid enhanced fruiting performance by increasing the total yield and number of large berries (≥25 mm). Future prospects for this study include establishment of an optimal structure of spruce fiber substrate suitable for strawberry production and development of the fertigation strategy optimized for the new growing media.
Sammendrag
Potato contributes highly to the global food security. It is predicted that the production of this crop will be negatively affected by future climatic changes. Application of hydroponics for table potato production can mitigate crop loss in highly vulnerable regions. A preliminary small-scale case-study was performed to test theoretical perspectives of hydroponic production of table potatoes in wood fiber by comparing different fiber types and fertigation strategies. Potatoes were also grown in the field to obtain a reference control. Hydroponic production of potato in a stand-alone wood fiber resulted in ca. 200% higher yield, when compared to standard soil cultivation. However, the quality of the tubers was slightly reduced (lower dry matter content). Productivity of table potatoes was affected by the growing medium and fertigation strategy. Production of potatoes in wood fiber is possible and, in the future, might complement the conventional production systems, or even become an important alternative in locations where in-field cultivation is not possible. Nevertheless, the effect of wood fiber properties and the applied fertigation strategy on yield potential and tuber quality should be further studied. Optimization of these factors will be a topic of a following full-scale research.
Forfattere
El Houssein Chouaib HarikSammendrag
One of the goals in adopting more sustainable agricultural practices is to reduce green-house-gas emissions from current practices by replacing fossil-fuel-based heavy machinery with lighter, electrical ones. In a not-so-distant scenario where a single farmer owns a fleet of small electrical tractors/robots that can operate in an autonomous/semi-autonomous manner, this will bring along some logistic challenges. It will be highly impractical that the farmer follows each time a given vehicle moves to the charging point to manually charge it. We present in this paper the design and implementation of an autonomous charging station to be used for that purpose. The charging station is a combination of a holonomic mobile platform and a collaborative robotic arm. Vision-based navigation and detection are used in order to plug the power cable from the wall-plug to the vehicle and back to the wall-plug again when the vehicle has recharged its batteries or reached the required level to pursue its tasks in the field. A decision-tree-based scheme is used in order to define the necessary pick, navigate, and plug sequences to fulfill the charging task. Communication between the autonomous charging station and the vehicle is established in order to make the whole process completely autonomous without any manual intervention. We present in this paper the charging station, the docking mechanism, communication scheme, and the deployed algorithms to achieve the autonomous charging process for agricultural electrical vehicles. We also present real experiments performed using the developed platform on an electrical robot-tractor.
Sammendrag
This study investigated the potential of in-season airborne hyperspectral imaging for the calibration of robust forage yield and quality estimation models. An unmanned aerial vehicle (UAV) and a hyperspectral imager were used to capture canopy reflections of a grass-legume mixture in the range of 450 nm to 800 nm. Measurements were performed over two years at two locations in Southeast and Central Norway. All images were subject to radiometric and geometric corrections before being processed to ortho-images, carrying canopy reflectance information. The data (n = 707) was split in two, using half the data for model calibration and the remaining half for validation. Several powered partial least squares regression (PPLSR) models were fitted to the reflectance data to estimate fresh (FM) and dry matter (DM) yields, as well as crude protein (CP), dry matter digestibility (DMD), neutral detergent fibre (NDF), and indigestible neutral detergent fibre (iNDF) content. Prediction performance of these models was compared with the prediction performance of simple linear regression (SLR) models, which were based on selected vegetation indices and plant height. The highest prediction accuracies for general models, based on the pooled data, were achieved by means of PPLSR, with relative root-mean-square errors of validation of 14.2% (2550 kg FM ha−1), 15.2% (555 kg DM ha−1), 11.7% (1.32 g CP 100 g−1 DM), 2.4% (1.71 g DMD 100 g−1 DM), 4.8% (2.72 g NDF 100 g−1 DM), and 12.8% (1.32 g iNDF 100 g−1 DM) for the prediction of FM, DM, CP, DMD, NDF, and iNDF content, respectively. None of the tested SLR models achieved acceptable prediction accuracies.
Forfattere
Xinbing Wang Yuxin Miao Rui Dong Hainie Zha Tingting Xia Zhichao Chen Krzysztof Kusnierek Guohua Mi Hong Sun Minzan LiSammendrag
Reliable and efficient in-season nitrogen (N) status diagnosis and recommendation methods are crucially important for the success of crop precision N management (PNM). The accuracy of these methods has been found to be influenced by soil properties, weather conditions, and crop management practices. It is important to effectively incorporate these variables to improve in-season N management. Machine learning (ML) methods are promising due to their capability of processing different types of data and modeling both linear and non-linear relationships. The objectives of this study were to (1) determine the potential improvement of in-season prediction of corn N nutrition index (NNI) and grain yield by combining soil, weather and management data with active sensor data using random forest regression (RFR) as compared with Lasso linear regression (LR) using similar data and simple regression (SR) models only using crop sensor data; and (2) to develop a new in-season side-dress N fertilizer recommendation strategy at eighth to ninth leaf stage (V8-V9) of corn developement using the RFR model. Twelve site-year experiments examining corn N rates and planting densities were conducted in Northeast China. The GreenSeeker sensor data and corn NNI were collected at V8-V9 stage, and grain yield was determined at the harvest stage (R6). The soil information was obtained at planting and the weather data was measured throughout the growing season. The results indicated that corn NNI and grain yield were better predicted by combining soil, weather and management information with GreenSeeker sensor data using RFR model (R2 = 0.86 and 0.79) and LR model (R2 = 0.85 and 0.76) as compared with only using GreenSeeker sensor data (R2 = 0.66 and 0.62–63) based on the test dataset. An innovative in-season side-dress N recommendation strategy was developed using the RFR grain yield prediction model to simulate corn grain yield responses to a series of side-dress N rates at V8-V9 stage. Based on these response curves, site-, and year-specific optimum side-dress N rates can be determined. The scenario analysis results indicated that this RFR model-based in-season N recommendation strategy could recommend side-dress N rates similar to those based on measured agronomic optimum N rate (AONR) or economic optimum N rate (EONR), with root mean square error (RMSE) of 17 kg ha−1 and relative error (RE) of 14–15 %. It is concluded that combining soil, weather and management information with crop sensor data using RFR can significantly improve both in-season corn NNI and grain yield prediction and N management, compared with the approach based only on crop sensor data. More studies are needed to further improve and evaluate this approach under diverse on-farm conditions.
Sammendrag
The rapidly expanding field of machine learning (ML) provides many methodological opportunities which match very well with the needs and challenges of hydrological research. Due to extended measurement networks, more frequent automatic measurements of hydrological variables, and not the least increasing use of remote sensing products, the era of big data surely has arrived in hydrology. Process-based models are usually developed for certain spatiotemporal scales, not fitting easily to the scope of the new datasets. Automatic methods that learn patterns and generalizations have been demonstrated to be superior in many applications. The chapter provides an overview of some of the most important machine learning algorithms which have been used in the hydrological literature. It will be shown that there is no single best method among them, but instead a spectrum of methods should be utilized, from highly flexible ones to more parsimonious learning methods, depending on the specific hydrological application, research question, and data availability. Most machine learning techniques require a calibration and a validation dataset for training. As these data are usually correlated in time and space, the problem of bias-variance tradeoff arises will be discussed as a simple example. The presentation of ML algorithms, roughly following chronological order, is discussed starting with artificial neural networks through support vector machines to gradient boosting machines. As data streams increase, these and other machine learning techniques will play an ever more important role in hydrology.
Forfattere
Junjun Lu Yuxin Miao Wei Shi Jingxin Li Xiaoyi Hu Zhichao Chen Xinbing Wang Krzysztof KusnierekSammendrag
RapidSCAN is a portable active canopy sensor with red, red-edge, and near infrared spectral bands. The objective of this study is to develop and evaluate a RapidSCAN sensor-based precision nitrogen (N) management (PNM) strategy for high-yielding rice in Northeast China. Six rice N rate experiments were conducted from 2014 to 2016 at Jiansanjiang Experiment Station of China Agricultural University in Northeast China. The results indicated that the sensor performed well for estimating rice yield potential (YP0) and yield response to additional N application (RIHarvest) at the stem elongation stage using normalized difference vegetation index (NDVI) (R2 = 0.60–0.77 and relative error (REr) = 6.2–8.0%) and at the heading stage using normalized difference red edge (NDRE) (R2 = 0.70–0.82 and REr = 7.3–8.7%). A new RapidSCAN sensor-based PNM strategy was developed that would make N recommendations at both stem elongation and heading growth stages, in contrast to previously developed strategy making N recommendation only at the stem elongation stage. This new PNM strategy could save 24% N fertilizers, and increase N use efficiencies by 29–35% as compared to Farmer N Management, without significantly affecting the rice grain yield and economic returns. Compared with regional optimum N management, the new PNM strategy increased 4% grain yield, 3–10% N use efficiencies and 148 $ ha−1 economic returns across years and varieties. It is concluded that the new RapidSCAN sensor-based PNM strategy with two in-season N recommendations using NDVI and NDRE is suitable for guiding in-season N management in high-yield rice management systems. Future studies are needed to evaluate this RapidSCAN sensor-based PNM strategy under diverse on-farm conditions, as well as to integrate it into high-yield rice management systems for food security and sustainable development.
Forfattere
Jiangsan Zhao Dmitry Kechasov Boris Rewald Gernot Bodner Michel Verheul Nicholas Clarke Jihong Liu ClarkeSammendrag
Hyperspectral imaging has many applications. However, the high device costs and low hyperspectral image resolution are major obstacles limiting its wider application in agriculture and other fields. Hyperspectral image reconstruction from a single RGB image fully addresses these two problems. The robust HSCNN-R model with mean relative absolute error loss function and evaluated by the Mean Relative Absolute Error metric was selected through permutation tests from models with combinations of loss functions and evaluation metrics, using tomato as a case study. Hyperspectral images were subsequently reconstructed from single tomato RGB images taken by a smartphone camera. The reconstructed images were used to predict tomato quality properties such as the ratio of soluble solid content to total titratable acidity and normalized anthocyanin index. Both predicted parameters showed very good agreement with corresponding “ground truth” values and high significance in an F test. This study showed the suitability of hyperspectral image reconstruction from single RGB images for fruit quality control purposes, underpinning the potential of the technology—recovering hyperspectral properties in high resolution—for real-world, real time monitoring applications in agriculture any beyond.
Forfattere
Xinbing Wang Yuxin Miao Rui Dong Zhichao Chen Krzysztof Kusnierek Guohua Mi David J. MullaSammendrag
The dynamic interactions between soil, weather and crop management have considerable influences on crop yield within a region, and should be considered in optimizing nitrogen (N) management. The objectives of this study were to determine the influence of soil type, weather conditions and planting density on economic optimal N rate (EONR), and to evaluate the potential benefits of site-specific N management strategies for maize production. The experiments were conducted in two soil types (black and aeolian sandy soils) from 2015 to 2017, involving different N rates (0 to 300 kg ha−1) with three planting densities (55,000, 70,000, and 85,000 plant ha−1) in Northeast China. The results showed that the average EONR was higher in black soil (265 kg ha−1) than in aeolian sandy soil (186 kg ha−1). Conversely, EONR showed higher variability in aeolian sandy soil (coefficient of variation (CV) = 30%) than in black soil (CV = 10%) across different weather conditions and planting densities. Compared with farmer N rate (FNR), applying soil-specific EONR (SS-EONR), soil- and year-specific EONR (SYS-EONR) and soil-, year-, and planting density-specific EONR (SYDS-EONR) would significantly reduce N rate by 25%, 30% and 38%, increase net return (NR) by 155 $ ha−1, 176 $ ha−1, and 163 $ ha−1, and improve N use efficiency (NUE) by 37–42%, 52%, and 67–71% across site-years, respectively. Compared with regional optimal N rate (RONR), applying SS-EONR, SYS-EONR and SYDS-EONR would significantly reduce N application rate by 6%, 12%, and 22%, while increasing NUE by 7–8%, 16–19% and 28–34% without significantly affecting yield or NR, respectively. It is concluded that soil-specific N management has the potential to improve maize NUE compared with both farmer practice and regional optimal N management in Northeast China, especially when each year’s weather condition and planting density information is also considered. More studies are needed to develop practical in-season soil (site)-specific N management strategies using crop sensing and modeling technologies to better account for soil, weather and planting density variation under diverse on-farm conditions.
Forfattere
Hainie Zha Yuxin Miao Tiantian Wang Yue Li Jing Zhang Weichao Sun Zhengqi Feng Krzysztof KusnierekSammendrag
Optimizing nitrogen (N) management in rice is crucial for China’s food security and sustainable agricultural development. Nondestructive crop growth monitoring based on remote sensing technologies can accurately assess crop N status, which may be used to guide the in-season site-specific N recommendations. The fixed-wing unmanned aerial vehicle (UAV)-based remote sensing is a low-cost, easy-to-operate technology for collecting spectral reflectance imagery, an important data source for precision N management. The relationships between many vegetation indices (VIs) derived from spectral reflectance data and crop parameters are known to be nonlinear. As a result, nonlinear machine learning methods have the potential to improve the estimation accuracy. The objective of this study was to evaluate five different approaches for estimating rice (Oryza sativa L.) aboveground biomass (AGB), plant N uptake (PNU), and N nutrition index (NNI) at stem elongation (SE) and heading (HD) stages in Northeast China: (1) single VI (SVI); (2) stepwise multiple linear regression (SMLR); (3) random forest (RF); (4) support vector machine (SVM); and (5) artificial neural networks (ANN) regression. The results indicated that machine learning methods improved the NNI estimation compared to VI-SLR and SMLR methods. The RF algorithm performed the best for estimating NNI (R2 = 0.94 (SE) and 0.96 (HD) for calibration and 0.61 (SE) and 0.79 (HD) for validation). The root mean square errors (RMSEs) were 0.09, and the relative errors were <10% in all the models. It is concluded that the RF machine learning regression can significantly improve the estimation of rice N status using UAV remote sensing. The application machine learning methods offers a new opportunity to better use remote sensing data for monitoring crop growth conditions and guiding precision crop management. More studies are needed to further improve these machine learning-based models by combining both remote sensing data and other related soil, weather, and management information for applications in precision N and crop management.
Forfattere
Zhichao Chen Yuxin Miao Junjun Lu Lan Zhou Yue Li Hongyan Zhang Weidong Lou Zheng Zhang Krzysztof Kusnierek Changhua LiuSammendrag
Improving nitrogen (N) management of small-scale farming systems in developing countries is crucially important for food security and sustainable development of world agriculture, but it is also very challenging. The N Nutrition Index (NNI) is a reliable indicator for crop N status, and there is an urgent need to develop an effective method to non-destructively estimate crop NNI in different smallholder farmer fields to guide in-season N management. The eBee fixed-wing unmanned aerial vehicle (UAV)-based remote sensing system, a ready-to-deploy aircraft with a Parrot Sequoia+ multispectral camera onboard, has been used for applications in precision agriculture. The objectives of this study were to (i) determine the potential of using fixed-wing UAV-based multispectral remote sensing for non-destructive estimation of winter wheat NNI in different smallholder farmer fields across the study village in the North China Plain (NCP) and (ii) develop a practical strategy for village-scale winter wheat N status diagnosis in small scale farming systems. Four plot experiments were conducted within farmer fields in 2016 and 2017 in a village of Laoling County, Shandong Province in the NCP for evaluation of a published critical N dilution curve and for serving as reference plots. UAV remote sensing images were collected from all the fields across the village in 2017 and 2018. About 150 plant samples were collected from farmer fields and plot experiments each year for ground truthing. Two indirect and two direct approaches were evaluated for estimating NNI using vegetation indices (VIs). To facilitate practical applications, the performance of three commonly used normalized difference VIs were compared with the top performing VIs selected from 59 tested indices. The most practical and stable method was using VIs to calculate N sufficiency index (NSI) and then to estimate NNI non-destructively (R2 = 0.53–0.56). Using NSI thresholds to diagnose N status directly was quite stable, with a 57–59% diagnostic accuracy rate. This strategy is practical and least affected by the choice of VIs across fields, varieties, and years. This study demonstrates that fixed-wing UAV–based remote sensing is a promising technology for in-season diagnosis of winter wheat N status in smallholder farmer fields at village scale. The considerable variability in local soil conditions and crop management practices influenced the overall accuracy of N diagnosis, so more studies are needed to further validate and optimize the reported strategy and consecutively develop practical UAV remote sensing–based in-season N recommendation methods.
Sammendrag
In this paper, we present a novel method for obstacle avoidance designed for a nonholonomic mobile robot. The method relies on light detection and ranging (LiDAR) readings, which are mapped into a polar coordinate system. Obstacles are taken into consideration when they are within a predefined radius from the robot. A central part of the approach is a new Heading Weight Function (HWF), in which the beams within the aperture angle of the LiDAR are virtually weighted in order to generate the best trajectory candidate for the robot. The HWF is designed to find a solution also in the case of a local-minima situation. The function is coupled with the robot’s controller in order to provide both linear and angular velocities. We tested the method both by simulations in a digital environment with a range of different static obstacles, and in a real, experimental environment including static and dynamic obstacles. The results showed that when utilizing the novel HWF, the robot was able to navigate safely toward the target while avoiding all obstacles included in the tests. Our findings thus show that it is possible for a robot to navigate safely in a populated environment using this method, and that sufficient efficiency in navigation may be obtained without basing the method on a global planner. This is particularly promising for navigation challenges occurring in unknown environments where models of the world cannot be obtained.
Forfattere
Trygve Utstumo Frode Urdal Anders Brevik Jarle Dørum Jan Netland Øyvind Overskeid Therese With Berge Jan Tommy GravdahlSammendrag
Vegetables and other row-crops represent a large share of the agricultural production. There is a large variation in crop species, and a limited availability in specialized herbicides. The robot presented here utilizes systematic growing techniques to navigate and operate in the field. By the use of machine vision it separates seeded vegetable crops from weed. Each weed within the row is treated with individual herbicide droplets, without affecting the crop. This results in a significant reduction in herbicide use, and allows for the use of herbicides that would otherwise harm the crop. The robot is tailored to this purpose with cost, maintainability, efficient operation and robustness in mind. The three-wheeled design is unconventional, and the design maintains maneuverability and stability with the benefit of reduced weight, complexity and cost. Indoor pot trials with four weed species demonstrated that the Drop-on-Demand system (DoD) could control the weeds with as little as 7.6 μg glyphosate or 0.15 μg iodosulfuron per plant. The results also highlight the importance of liquid characteristics for droplet stability and leaf retention properties. The common herbicide glyphosate had no effect unless mixed with suitable additives. A field trial with the robot was performed in a carrot field, and all the weeds were effectively controlled with the DoD system applying 5.3 μg of glyphosate per droplet. The robot and DoD system represent a paradigm shift to the environmental impact and health risks of weed control, while providing a valuable tool to the producers.
Sammendrag
The key factor for autonomous navigation is efficient perception of the surroundings,while being able to move safely from an initial to a final point. We deal in this paper with a wheeled mobile robot working in a GPS-denied environment typical for a greenhouse. The Hector Simultaneous Localization and Mapping (SLAM) approach is used in order to estimate the robots’ pose using a LIght Detection And Ranging (LIDAR) sensor. Waypoint following and obstacle avoidance are ensured by means of a new artificial potential field (APF) controller presented in this paper. The combination of the Hector SLAMand the APF controller allows themobile robot to performperiodic tasks that require autonomous navigation between predefined waypoints. It also provides themobile robot with a robustness to changing conditions thatmay occur inside the greenhouse, caused by the dynamic of plant development through the season. In this study, we show that the robot is safe to operate autonomously with a human presence, and that in contrast to classical odometrymethods, no calibration is needed for repositioning the robot over repetitive runs. We include here both hardware and software descriptions, as well as simulation and experimental results.
Forfattere
Therese With BergeSammendrag
Det er ikke registrert sammendrag
Sammendrag
Today’s modern precision agriculture applications have a huge demand for data with high spatial and temporal resolution. This leads to the need of unmanned aerial vehicles (UAV) as sensor platforms providing both, easy use and a high area coverage. This study shows the successful development of a prototype hybrid UAV for practical applications in precision agriculture. The UAV consists of an off-the-shelf fixed-wing fuselage, which has been enhanced with multi-rotor functionality. It was programmed to perform pre-defined waypoint missions completely autonomously, including vertical take-off, horizontal flight, and vertical landing. The UAV was tested for its return-to-home (RTH) accuracy, power consumption and general flight performance at different wind speeds. The RTH accuracy was 43.7 cm in average, with a root-mean-square error of 39.9 cm. The power consumption raised with an increase in wind speed. An extrapolation of the analysed power consumption to conditions without wind resulted in an estimated 40 km travel range, when we assumed a 25 % safety margin of remaining battery capacity. This translates to a maximal area coverage of 300 ha for a scenario with 18 m/s airspeed, 50 minutes flight time, 120 m AGL altitude, and a desired 70 % of image side-lap and 85 % forward-lap. The ground sample distance with an in-built RGB camera was 3.5 cm, which we consider sufficient for farm-scale mapping missions for most precision agriculture applications.
Sammendrag
In this study, we investigated the potential of airborne imaging spectroscopy for in-season grassland yield estimation. We utilized an unmanned aerial vehicle and a hyperspectral imager to measure radiation, ranging from 455 to 780 nm. Initially, we assessed the spectral signature of five typical grassland species by principal component analysis, and identified a distinct reflectance difference, especially between the erectophil grasses and the planophil clover leaves. Then, we analyzed the reflectance of a typical Norwegian sward composition at different harvest dates. In order to estimate yields (dry matter, DM), several powered partial least squares (PPLS) regression and linear regression (LR) models were fitted to the reflectance data and prediction performance of these models were compared with that of simple LR models, based on selected vegetation indices and plant height. We achieved the highest prediction accuracies by means of PPLS, with relative errors of prediction from 9.1 to 11.8% (329 to 487 kg DM ha−1) for the individual harvest dates and 14.3% (558 kg DM ha−1) for a generalized model.
Sammendrag
The success of precision agriculture relies largely on our ability to identify how the plants’ growth limiting factors vary in time and space. In the field, several stress factors may occur simultaneously, and it is thus crucial to be able to identify the key limitation, in order to decide upon the correct contra-action, e.g., herbicide application. We performed a pot experiment, in which spring wheat was exposed to water shortage, nitrogen deficiency, weed competition (Sinapis alba L.) and fungal infection (Blumeria graminis f. sp. tritici) in a complete, factorial design. A range of sensor measurements were taken every third day from the two-leaf stage until booting of the wheat (BBCH 12 to 40). Already during the first 10 days after stress induction (DAS), both fluorescence measurements and spectral vegetation indices were able to differentiate between non-stressed and stressed wheat plants exposed to water shortage, weed competition or fungal infection. This meant that water shortage and fungal infection could be detected prior to visible symptoms. Nitrogen shortage was detected on the 11–20 DAS. Differentiation of more than one stress factors with the same index was difficult.
Sammendrag
Det er ikke registrert sammendrag
Sammendrag
Det er ikke registrert sammendrag
Sammendrag
It has been long known that thermal imaging may be used to detect stress (e.g. water and nutrient deficiency) in growing crops. Developments in microbolometer thermal cameras, such as the introduction of imaging arrays that may operate without costly active temperature stabilization, have vitalized the interest in thermal imaging for crop measurements. In this study, we have focused on the challenges occurring when temperature stabilization is omitted, including the effects of focal-plane-array (FPA) temperature, camera settings and the environment in which the measurements are performed. Further, we have designed and tested models for providing thermal response from an analog LWIR video signal (typical output from low-cost microbolometer thermal cameras). Finally, we have illustrated and discussed challenges which typically occur under practical use of thermal imaging of crops, by means of three cereal showcases, including proximal and remotely based (UAV) data acquisition. The results showed that changing FPA temperature greatly affected the measurements, and that wind and irradiance also appeared to affect the temperature dynamics considerably. Further, we found that adequate settings of camera gain and offset were crucial for obtaining a reliable result. The model which was considered best in terms of transforming video signals into thermal response data included information on camera FPA temperature, and was based on a priori calibrations using a black-body radiation source under controlled conditions. Very good calibration (r2>0.99, RMSE=0.32°C, n=96) was obtained for a target temperature range of 15-35°C, covering typical daytime crop temperatures in the growing season. However, the three showcases illustrated, that under practical conditions, more factors than FPA temperature may need to be corrected for. In conclusion, this study shows that thermal data acquisition by means of an analog, uncooled thermal camera may represent a possible, cost-efficient method for the detection of crop stress, but appropriate corrections of disturbing factors are required in order to obtain sufficient accuracy.
Forfattere
JC. Streibig J. Rasmussen D. Andùjar C. Andreasen Therese With Berge D. Chachalis T. Dittmann Gerhard Gerhardsen T. M. Giselsson P. Hamouz C. Jaeger-Hansen K. Jensen R.N. Jørgensen M. Keller M. Laursen H.S Midtiby J. Nielsen S. Muller H. Nordmeyer G. Peteinatos A Papadopoulos J. Svensgaard M. Weis S. ChristensenSammendrag
Det er ikke registrert sammendrag
Sammendrag
Det er ikke registrert sammendrag
Sammendrag
Det er ikke registrert sammendrag
Sammendrag
Det er ikke registrert sammendrag
Sammendrag
Arable weeds are generally distributed in patches, while herbicides are applied uniformly. Herbicides can be saved if only the patches are sprayed, i.e. patch spraying (PS). Bottlenecks for cost-effective PS are weed monitoring technology and valid technology-based decision rules for PS (thresholds). The novel machine vision algorithm Weedcer has been developed as an efficient weed monitoring tool for PS. Weedcer estimates the proportions of young weed leaves and cereal leaves in high resolution red–green–blue images. We conducted field trials to test relative weed cover (RWC) and relative mayweed cover (RMC) estimated by Weedcer as decision rules for PS. RWC is the total weed cover divided by the total plant cover and RMC is the mayweed cover divided by the total plant cover. The main criterion for evaluation and basis of these thresholds was the measured grain yield. Images (about 0.06-m2) were acquired with a GPS guided autonomous field robot in spring, the normal time for spraying seed-propagated broadleaf weeds in both winter – and spring cereals in Norway. Three map-based trials (weed monitoring and spraying in two separate operations) showed that mean RWC per management unit (12.0 × 12.5-m) was generally adequate. In winter wheat heavily infested with scentless mayweed (Tripleurospermum inodorum (L.) Sch.Bip.) and/or scented mayweed (Matricaria recutita L.), the mean RMC per management unit was more adequate. Progress during the project allowed three additional trials conducted in real-time (weed monitoring and spraying in the same operation). These were conducted with the robot in spring cereals, and showed that a weighted moving average of RWC per image was adequate. The sprayed and unsprayed management units in these trials were minimum 3.0 × 3.0-m and 0.5 × 3.0-m, respectively. Results indicated that the Weedcer-based thresholds should be lower in wheat (Triticum aestivum) than in barley (Hordeum vulgare).
Sammendrag
Det er ikke registrert sammendrag
Sammendrag
Det er ikke registrert sammendrag
Sammendrag
Det er ikke registrert sammendrag
Forfattere
Audun KorsæthSammendrag
Det er ikke registrert sammendrag
Sammendrag
Lack of automatic weed detection tools has hampered the adoption of site-specific weed control in cereals. An initial object-oriented algorithm for the automatic detection of broad-leaved weeds in cereals developed by SINTEF ICT (Oslo, Norway) was evaluated. The algorithm ("WeedFinder") estimates total density and cover of broad-leaved weed seedlings in cereal fields from near-ground red-green-blue images. The ability of "WeedFinder" to predict 'spray'/'no spray' decisions according to a previously suggested spray decision model for spring cereals was tested with images from two wheat fields sown with the normal row spacing of the region, 0.125 m. Applying the decision model as a simple look-up table, "WeedFinder" gave correct spray decisions in 65-85% of the test images. With discriminant analysis, corresponding mean rates were 84-90%. Future versions of "WeedFinder" must be more accurate and accommodate weed species recognition.
Sammendrag
A possible cost-effective real-time patch spraying implementation against seed-propagated broad-leaved weeds in cereals is a camera mounted in front of the tractor taking images at feasible distances in the direction of travel, on-board image analysis software and entire boom switched on and off. To assess this implementation, manual weed counts (0.25 m(2) quadrats) in a 1.5 m x 2 m grid, were used to simulate camera outputs. Each quadrat was classified into 'spray' and 'not spray' decisions based on a threshold model, and the resulting map defined the 'ground truth'. Subsequently, 'on/off' spraying at larger control areas where sizes were given by the boom width and image distance, and spraying decision controlled by weed status at the single quadrat simulating the camera's view, were simulated. These coarser maps were compared with 'ground truth', to estimate mapping error (area above threshold not sprayed), spraying error (area below threshold sprayed), total error (sum of mapping and spraying error) and the herbicide reduction. Three levels of the threshold model were tested. Results were used to fit models that predict errors from boom width and image distance. Size of control area did not on average affect the magnitude of the simulated herbicide reductions, but the bigger the control area the higher the risk that the simulated herbicide reduction deviate from the reduction in 'ground truth'. Mean simulated herbicide reductions were 42-59%, depending on threshold level. Only minor differences due to threshold level were seen for mean mapping and spraying errors at given spraying resolutions. Using original threshold level and image distance 2 m, predicted total errors for boom widths 2 m, 6 m, 20 m and 40 m would be 6%, 10%, 16% and 17%, respectively. Results indicate that control area should not exceed about 10 m 2 if acceptable total error is maximum 10%.
Forfattere
Audun KorsæthSammendrag
A 3.3 ha field experiment with tile-drained plots was established in 1988/89 at the Research Centre of the Arable Crops Division in central southeast Norway (60°42"N, 10°51"E, altitude 250 m). Six cropping systems, each with 2 replicates, are practiced on twelve 1.8 ha blocks, arranged in a randomised complete block design. During the first 10 years, the experiment provided data for many studies covering a wide range of topics. Some adjustments were made to the experimental treatments in 2000. The experiment now comprises three arable systems ("old-fashioned" and "modern" conventional arable cropping, and organic arable cropping with green manure as its only nutrient input) and three mixed dairy systems ("modern" conventional production of both arable and forage crops with 50% grass-clover ley, and organic production of both arable and forage crops with either 50 or 75% grass-clover ley in the rotation, all with farmyard manure). In this study, yields and N leaching/runoff losses are presented for the six agrohydrological years (May-April) 2001-2006. Results are discussed in relation to N use efficiency and sustainability of the systems.
Forfattere
Therese With Berge Haldor Fykse Are H. AastveitSammendrag
A major obstacle to patch spraying of broad-leaved weeds in cereals is a cost-effective method to assess within-field heterogeneity of the weed population. One method could be a camera mounted in front of the spraying vehicle, online image analysis, and field sprayer shifting between "on" and "off" as the predefined weed damage threshold level is reached. Because such a camera will capture a very limited area (
Forfattere
Audun KorsæthSammendrag
Det er ikke registrert sammendrag
Sammendrag
Det er ikke registrert sammendrag
Forfattere
Audun KorsæthSammendrag
Det er ikke registrert sammendrag
Sammendrag
Spring barley was grown for four years (2001-2004) in field trials consisting of 20 replicate blocks at two sites on morainic soil in central SE Norway. Five N level treatments were used within each block: 0, 60, 90, 120 and 150 kg N ha-1. Regression analyses showed that a selection of soil properties could explain 55-96% of the spatial yield variation and 18-91% of the variation in yield response to N. A variable-rate strategy, accounting for variation between both years and replicate blocks (VRs+t), was compared with a strategy, which accounted for variation between years, and a uniform strategy, which did not account for any variation. The VRs+t strategy had the highest potential yield, apparent fertilizer recovery and net revenue (yield value minus N cost). Using the VRs+t strategy, even at sub-optimal N rates, would increase the profit of barley cropping as long as the increase in net revenue was at least 24 and 42% of the estimated potentials, respectively.
Forfattere
Audun KorsæthSammendrag
Apparent soil electrical conductivity (ECa) is a promising indicator for important soil physical and chemical properties. In this paper the method of measuring ECa to characterize within-field variability was tested on a clay soil in S Norway. A field survey was conducted with the Geonics EM38 on a 15 ha field, and 223 soil samples were taken. Most of the measured variables of the topsoil, except P-AL, total N and organic C, were significantly (p£0.05) correlated with ECa. Topsoil Mg-AL alone accounted for 75% of the variation in ECa. Subsoil pH and clay, silt and coarse sand contents were correlated with ECa. The clay content at both depths accounted for 63% of the variation in ECa. After grouping the data on the basis of measured ECa into classes with intervals of 2 mS/m, there were significant differences in soil properties (combinations of clay content, Mg-AL and K-HNO3) between 7 out of 9 classes.
Forfattere
Audun KorsæthSammendrag
Two hand-held spectro-radiometers were used to measure canopy reflectance from winter wheat and spring barley in two ongoing fertilizer experiments. Relations between measurements made in June and plant N content (June), above-ground biomass (June), yields and grain protein content (at harvest in August) were studied. For winter wheat, regression models predicted up to 55, 89, 88 and 28% of the variations in N-content, biomass, yields and protein content, respectively. For spring barley the corresponding predictions were 87, 96, 87 and 22%.
Sammendrag
.Precision agriculture (PA) may be defined as using the best available technologies to tailor soil and crop management to fit the specific conditions found within an agricultural field or tract (Johannsen, 2001). Knowledge about soil variation within fields is thus a prerequisite for optimum PA. The use of sensors which measure electrical conductivity (EC) has been introduced as a promising way of mapping within-field variation in soil properties. In this paper we present relationships found between EC and both clay content and ignition loss (SOM) of some morainic loam soils in SE Norway. Measurements of EC at Møystad (60°47"N, 11°10"E, altitude ca.150 m) correlated well with clay content. Despite the rather narrow range of clay content at Møystad (11-17%), EC measurements accounted for about 70% of the variation. The use of EM38 in the horizontal position gave the best prediction in the upper two layers, whereas measurements in the vertical position fitted best to data from the deepest layer (40-60 cm). This is reasonable, since the instrument has its deepest range in the vertical position. Ignition loss in the upper layer was 5-8% at Møystad. There was no significant relation between EC and ignition loss in the upper layer, when EC was measured with EM38 in the vertical position. When EC was measured horizontally, about 24% of the variation in ignition loss was reflected by the EC of the soil. One should, however, take into account that ignition loss and clay content were positively correlated with each other (r=0.382), so that the result may in fact have been due to variation in clay content. We also measured EC at Kise Research Station (60°46"N, 10°48"E, altitude ca.130 m), where we selected five points across a field with a particularly large gradient in ignition loss. Here EC correlated positively with clay content, but this was not statistically significant (R2=0.576, p=0.137). With ignition loss, however, EC showed a strong positive correlation (R2=0.878, p=0.019). Inclusion of both clay content and ignition loss in a two-predictor regression model, with EC as the dependent variable, showed that the conductivity measurements depended almost completely on clay content and ignition loss for the selected points at Kise (R2=0.981, p=0.019). However, the predictors were positively intercorrelated here as well (r=0.517), which may make the statistical approach questionable. We also admit that the number of data points was very low. Nevertheless, the results clearly illustrate the potential of EC measurements for mapping soil variation.
Forfattere
Audun KorsæthSammendrag
.Dense datasets are required to describe within field variation precisely. Sensor techniques appear to be promising to reduce labour, time and costs compared to traditional methods of sampling and analysis. Relationships found between soil apparent electrical conductivity (ECa) and available N, pH and soil moisture under spring barley on morainic loam in SE Norway are presented. Measurements were conducted in a 160 m long field trial, established in barley in 2002 at Kise Research Station (60°46"N, 10°48"E, 130 masl). The trial had 20 replicate blocks containing five N-level treatments (0, 60, 90, 120 and 150 kg N ha-1, given as calcium ammonium nitrate). Each plot was 1.5 x 8 m. Soil samples were taken from all five treatments in three selected blocks at 0-15 cm depth, shortly before fertilizing/sowing (10.05.02) and then at two week intervals until the beginning of July (23.05, 06.06, 20.06 and 04.07). Analyses comprised nitrate-N, ammonium-N, pH and water content. At each sampling, ECa was measured in the same plots, using a magnetic dipole soil conductivity meter (EM38, Geonics Ltd., Canada). The device was operated manually in both horizontal (EMh) and vertical (EMv) modes. Linear regressions showed that both EMh and EMv correlated well with the measured variables. All the relations were significant (p
Sammendrag
Two trials have been established to study within-field variation in responses to N fertilizer. Preliminary results showed a close relation between soil organic matter and the amount of mineralised N in soil in spring. Both trials displayed considerable variation in responses to N fertilizer, even thought the yields increased up to the highest N level used. On the basis of responses in individual replicates, it was estimated that varying fertilizer levels across the field would have been positive in one of the trials. The studies will be continued to find out whether the pattern of variation remains the same, and to study the role of various soil factors.
Prosjekter
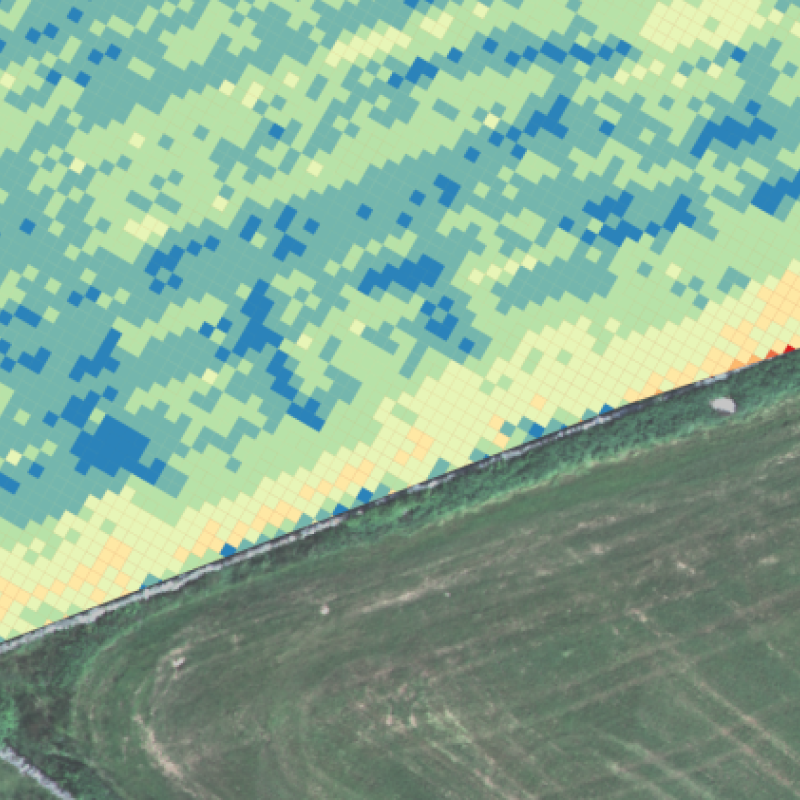
Divisjon for matproduksjon og samfunn
COPERNICUS - Jordbruk
Formålet med prosjektet er å ta i bruk satellitt-data fra Copernicus programmet for å utvikle rutiner og verktøy rettet inn mot jordbruksfaglige problemstillinger, og gjennom dette bidra med informasjon og råd til relevante aktører (bønder, rådgivere, jordbruksnæringa, kommuner, politikere og utdanningsinstitusjoner). Prosjektet skal dermed bidra til å forbedre dagens dyrkningspraksis, som gjennom en bedre utnyttelse av innsatsfaktorer som gjødsel og fôr også bidrar til å redusere klimaavtrykket til det norske jordbruket.
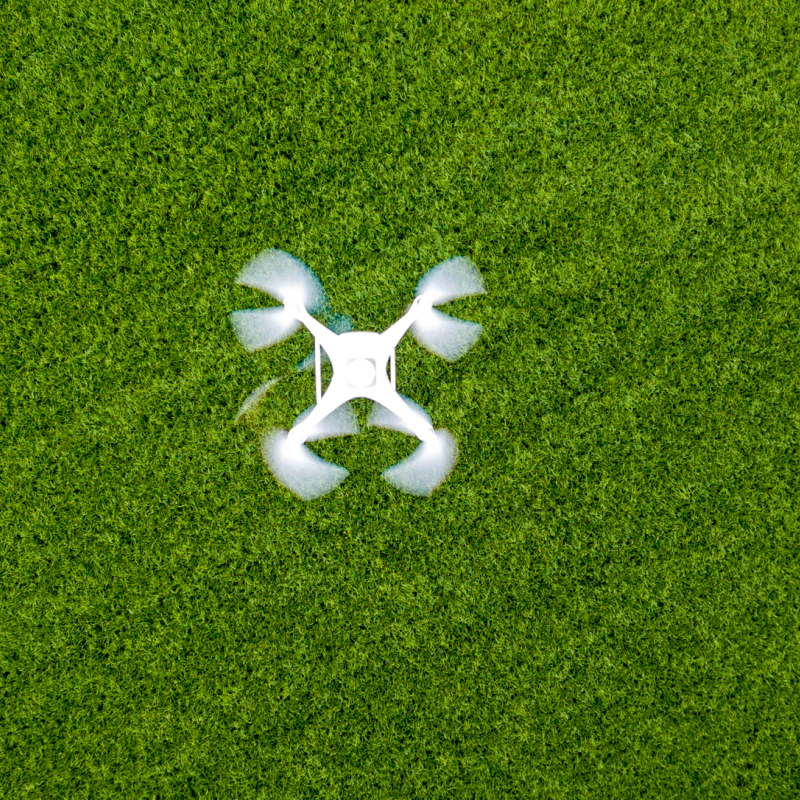
Divisjon for matproduksjon og samfunn
PRESIS
I PRESIS, et nytt femårig samarbeidsprosjekt, skal NIBIO være med å videreutvikle teknologiske løsninger for enda bedre presisjonsjordbruk, og samtidig sørge for at løsningene kommer ut i praksis til gårdene.
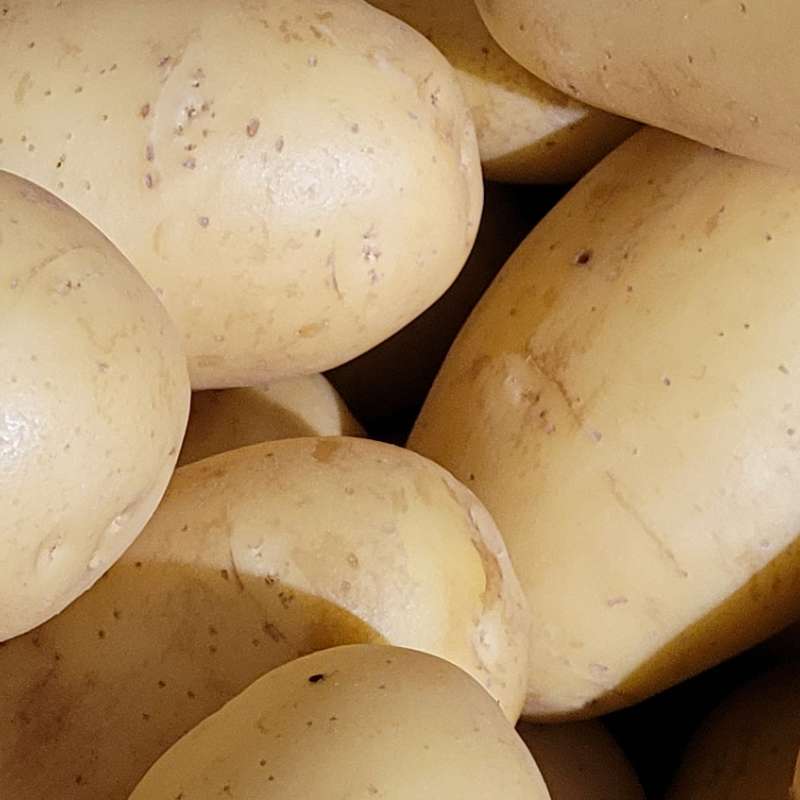
Divisjon for matproduksjon og samfunn
TEKNOPOTET - Ny teknologi for økt presisjon i produksjon og lagring av små matpoteter
Forbruket av matpoteter er i senere tid dreid mot en økt andel små matpoteter, såkalt delikatessepoteter. Hovedmålet for prosjektet er å utvikle ny kunnskap, teknologi og verktøy for økt presisjon i dyrking og lagring av slike småpoteter. Formålet er at markedet for småpoteter i størst mulig grad skal kunne dekkes av norske småpoteter med rett kvalitet. For at produksjonen skal være lønnsom må antall knoller per plante økes, knollene må ha rett størrelse og være mest mulig jevnstore, og lagringsstrategiene må tilpasses poteter som er små og pakkes tettere i kassene.
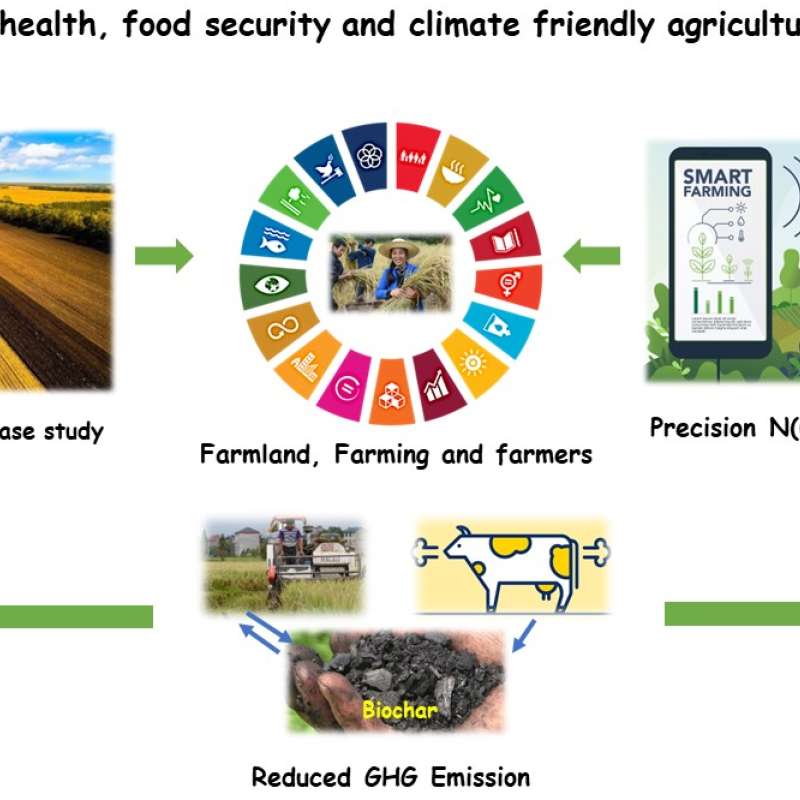
Divisjon for miljø og naturressurser
Sinograin III: Smart agricultural technology and waste-made biochar for food security, reduction of greenhouse gas (GHG) emission, and bio-and circular economy
The Sinograin III project’s overall objective is to contribute to the UN SDGs by widely implementing precision agriculture technologies and application of “waste-to-value” biochar products to achieve sustainable food production with minimized GHG emission, improve soil fertility and promote green growth/zero waste in modern agriculture in China.
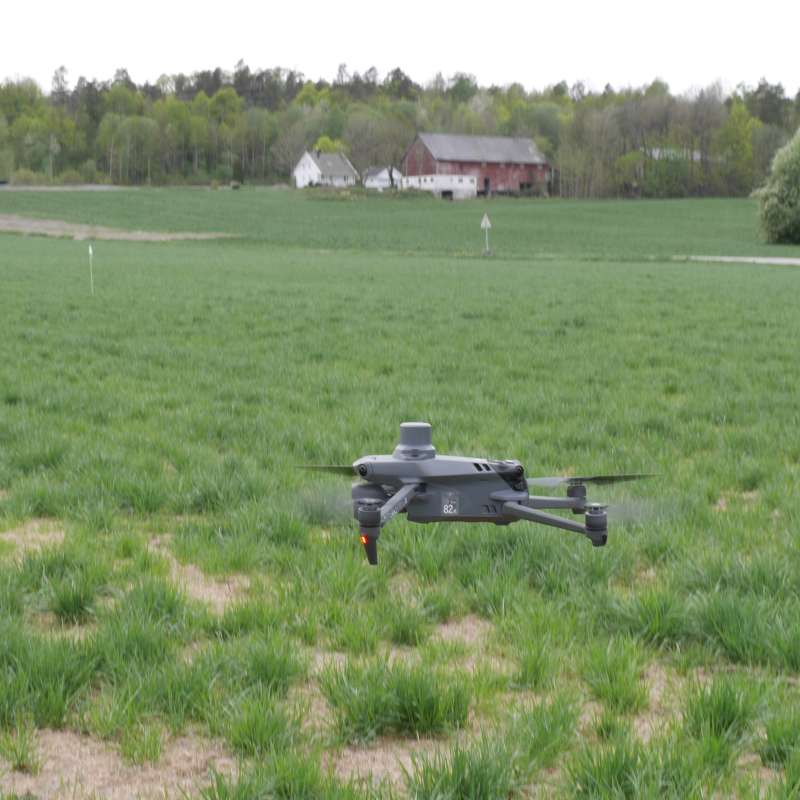
Divisjon for matproduksjon og samfunn
Presis gjødsling og vekstregulering av norske grasfrøenger ved hjelp av sensorteknologi (SmartSeed)
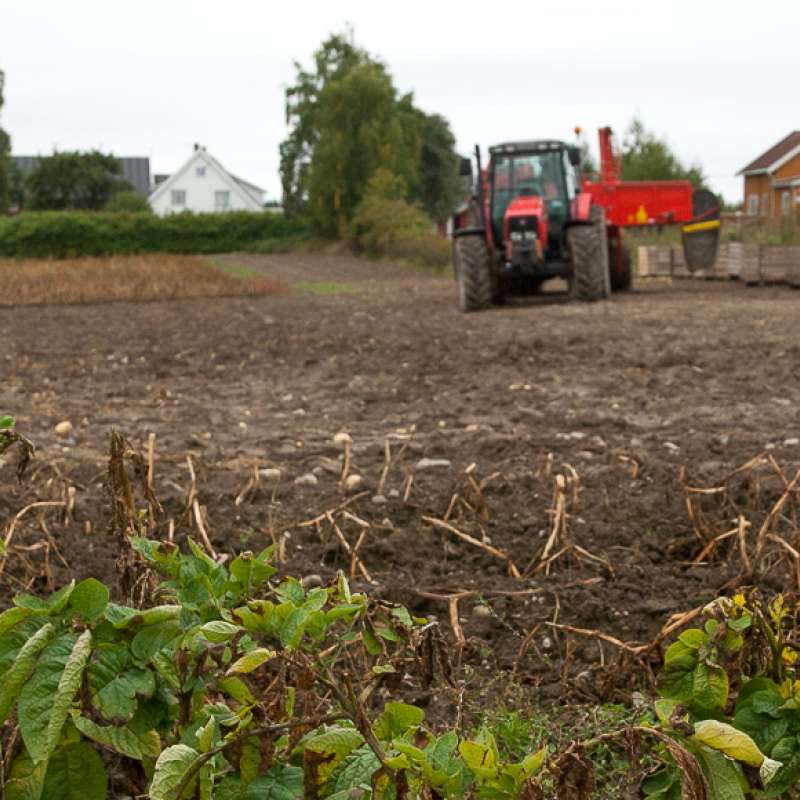
Divisjon for bioteknologi og plantehelse
SOLUTIONS: New solutions for potato canopy desiccation, control of weeds and runners in field strawberries & weed control in apple orchards
Efficient measures for weed control and similar challenges are vital to avoid crop loss in agriculture. National supply of food, feed and other agricultural products depends on each farmer’s success managing their fields and orchards. The recent loss of the herbicide diquat, and the potential ban on glyphosate, - both important tools for farmers -, raise a demand for new measures for vegetation control. Efficient alternatives to herbicides are also important tools in Integrated Pest Management (IPM). Norwegian growers need to document compliance to IPM since 2015 to ensure minimum hazards to health and environment from pesticide use.
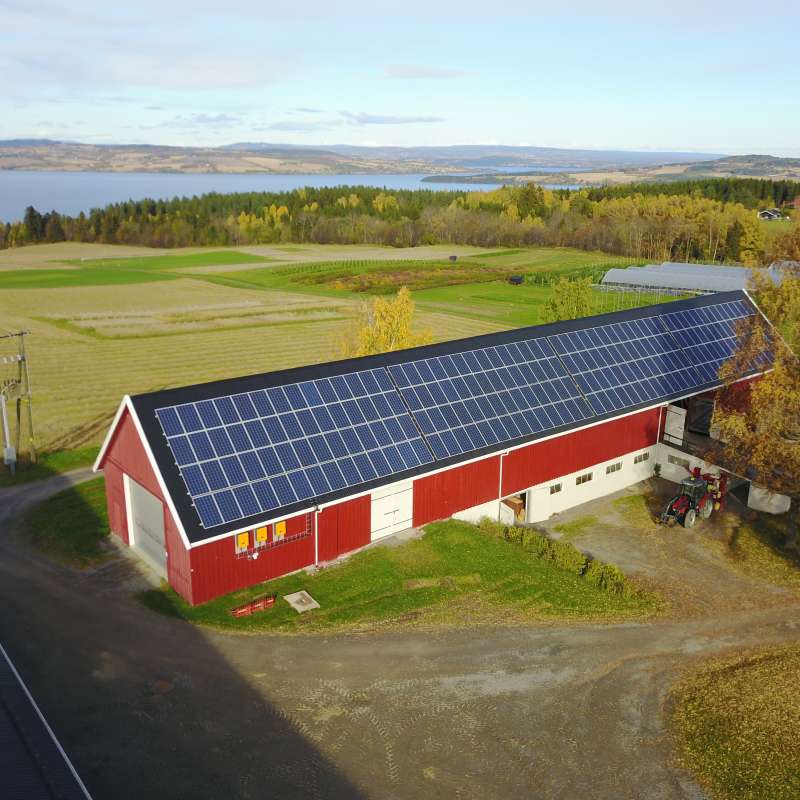
Divisjon for matproduksjon og samfunn
SolarFarm
SolarFarm - En systemstudie av hvordan solstrøm produsert på gårdsnivå kan drive elektriske og delvis selvstyrte farkoster i et presisjonsjordbruk med reduserte utslipp av klimagasser
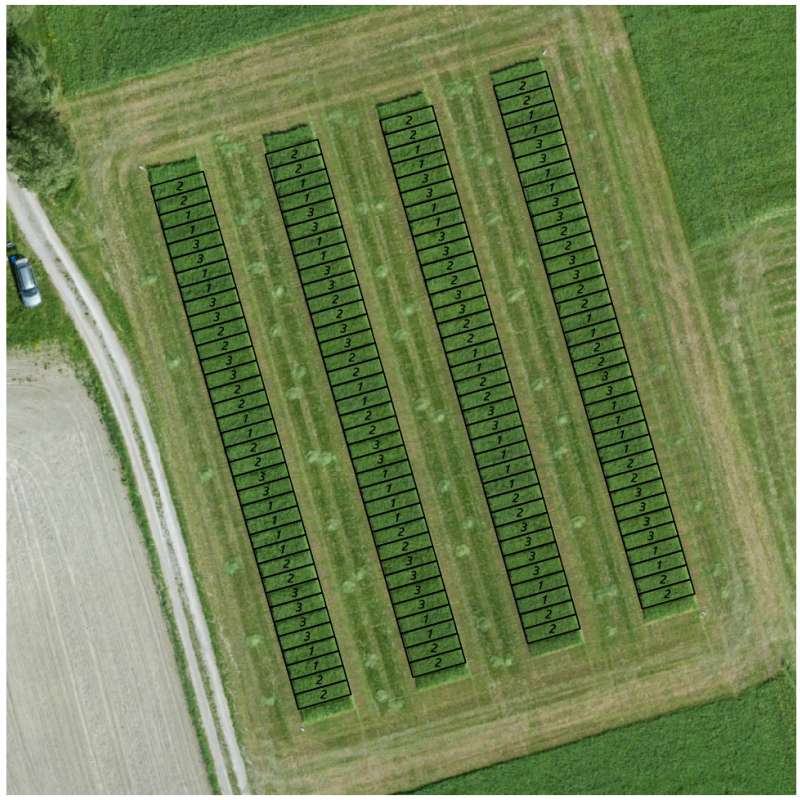
Divisjon for matproduksjon og samfunn
IMPRESS
IMPRESS - Økt presisjon og målretting i gjødsling og avlingsfremmende tiltak i grovfôrproduksjonen Hovedmålet med prosjektet er å heve avling og kvalitet i norsk grovfôrproduksjon gjennom å utvikle og tilpasse presisjonsteknologi, modeller og tiltak for stedsspesifikk bruk av innsatsfaktorer, og for identifikasjon og forbedring av lavtytende områder i enga
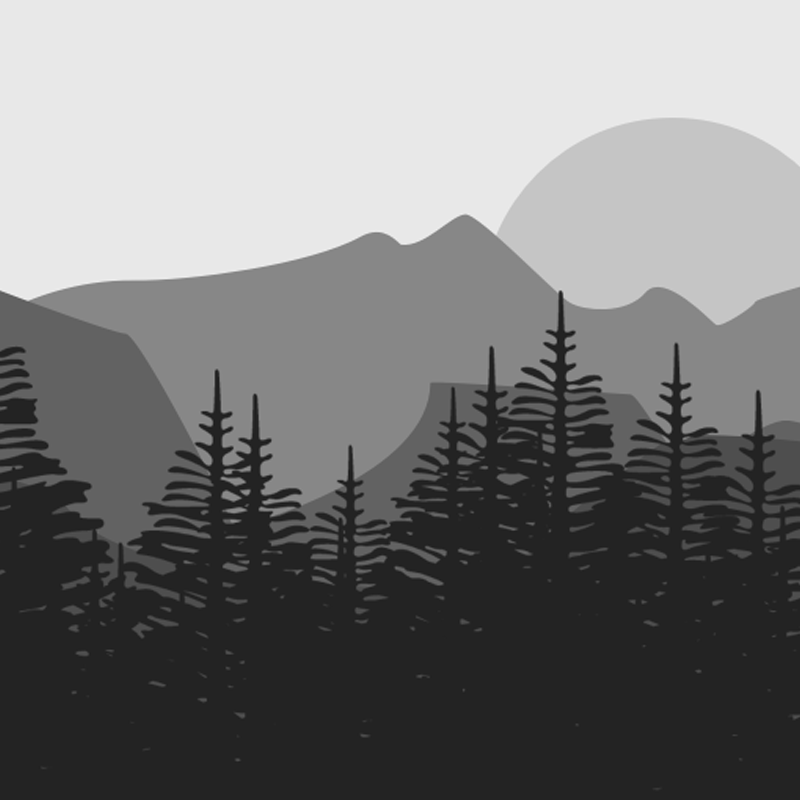
STRESSLESS
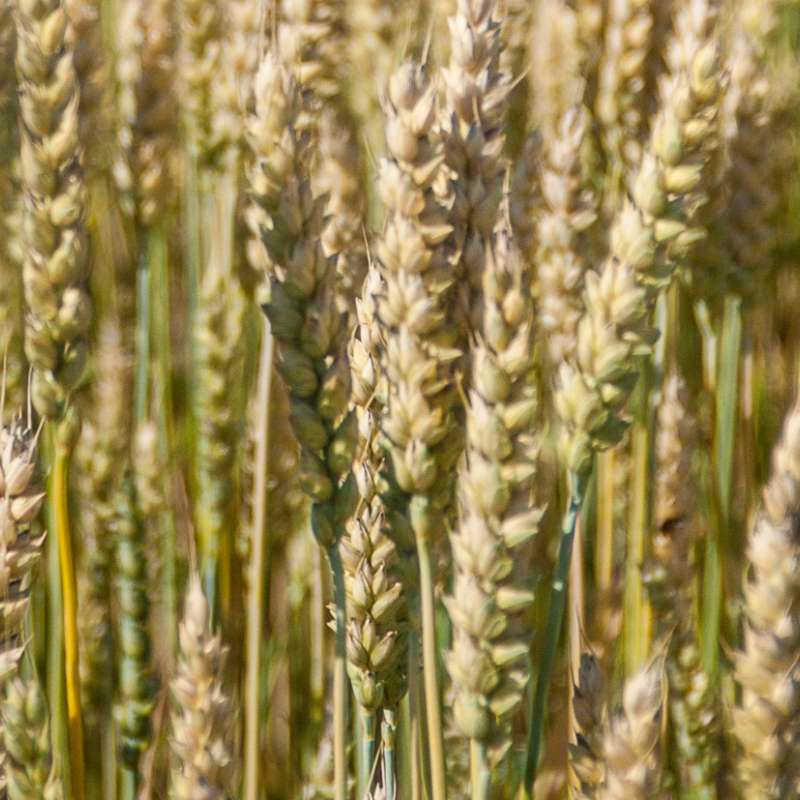
Divisjon for bioteknologi og plantehelse
QualityWheat - Norwegian wheat with optimized protein content and high baking quality
The Quality Wheat project aims to increase Norwegian wheat quality to achieve better utilization of Norwegian wheat, and thus meet the national goals of increased food production.