Presisjonsgjødsling
Foto: Kristian Rindal, NIBIO
Stor variasjon innen et skifte kan tilsi at det er mulig å oppnå gevinster ved bruk av presisjonsgjødsling.
Presisjonsgjødsling innebærer å tilpasse gjødslingen til plantenes spesifikke behov for næringsstoffer på ulike steder innen et skifte. Det krever både effektiv kartlegging av dette behovet og utstyr som kan tildele variabel mengde gjødsel.
Behovet for gjødsling av planter vil variere i et skifte basert på variasjoner i jordegenskaper, topografi, værforhold og mikroklima og påvirkning fra tidligere agronomisk praksis som drenering, jordpakking og vekstskifte. Gjennom presisjonsgjødsling forsøker vi å tilpasse oss behovene ved hjelp av variabel tildeling av næringsstoffer. Teknologi som GNSS, sensorer montert på plattformer som satellitter, droner eller bakkegående utstyr samt maskinlæring og kunstig intelligens brukes for å kartlegge den stedsspesifikke variasjonen i gjødslingsbehov.
Både vi ved Senter for Presisjonsjordbruk og andre forskningsmiljøer har vist at stedsspesifikk nitrogengjødsling øker bondens lønnsomhet og samtidig reduserer de negative miljøeffektene.
Vårt arbeid innen dette startet i 2001, med spesielt fokus på utfordringen med å identivisere faktisk nitrogenbehov når planten er utsatt for flere stressfaktorer samtidig. Vi har sett nærmere på stressfaktorer som omfatter for mye eller for lite vann, ugraskonkurranse og sykdomsangrep. Arbeidet har primært vært rettet mot korn og bær.
KONTAKTPERSON
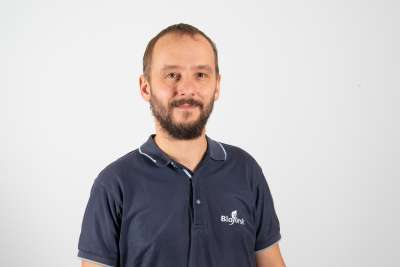
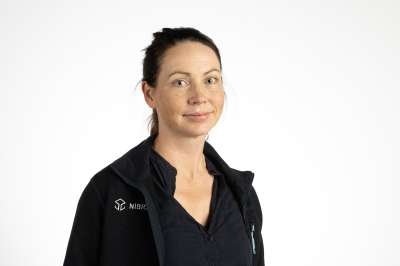
KONTAKTPERSON
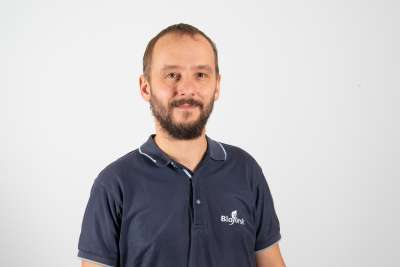
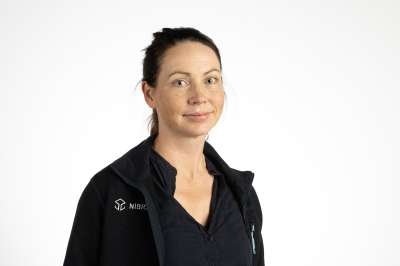
Publikasjoner
Abstract
Rapid methods allowing for non-destructive crop monitoring are imperative for accurate in-season nitrogen (N) status assessment and precision N management. The objectives of this paper were to (1) compare the performance of a leaf fluorescence sensor Dualex 4 and an active canopy reflectance sensor Crop Circle ACS-430 for estimating maize (Zea mays L.) N status indicators across growth stages; (2) evaluate the potential of N status prediction across growth stages using the reflectance parameters acquired from the canopy sensor at an early growth stage; and, (3) investigate the prospect of combining the active canopy sensor and leaf fluorescence sensor data to estimate N nutrition index (NNI) indirectly using a general model across growth stages. The results indicated that data from both sensors were closely related to NNI across stages. However, using the direct NNI estimation method, among the tested indices, only the N balance index (NBI) could diagnose N status satisfactorily, based on the Kappa statistics. The effect of growth stages on proximal sensing was reduced by incorporating the information of days after sowing. It was found that the leaf fluorescence sensor performed relatively better in estimating plant N concentration whereas the canopy reflectance sensor performed better in aboveground biomass estimation. Their combination significantly improved the reliability of N diagnosis, including NNI prediction. In addition, the study confirmed that N status can be assessed by predicting aboveground biomass at the later stages using the canopy reflectance measurements at an early stage. Furthermore, the integrated NBI was verified to be a more robust and sensitive N status indicator than the chlorophyll concentration index. It is concluded that combining active canopy sensor data, of an early growth stage (e.g. V8), with leaf fluorescence sensor data, modified using days after sowing, can improve the accuracy of corn N status diagnosis across growth stages.
Abstract
Accurate and non-destructive diagnosis of crop nitrogen (N) surplus and deficit status based on N nutrition index (NNI) is crucially important for the success of precision N management to improve N use efficiency (NUE) and reduce negative environmental impacts. However, due to the variability of the reflectance data obtained from different active crop sensors and complexity of the environmental and management conditions for regional applications, accurate determination of crop N status and topdressing N rate only using active canopy sensor data is very challenging. The objectives of this study were to (1) develop an in-season N status diagnosis and recommendation model based on NNI prediction using multi-source data fusion with machine learning, and (2) evaluate the accuracy of N diagnosis and recommendation in terms of rice yield and NUE under diverse on-farm conditions. Thirty plot experiments and thirteen on-farm experiments were conducted in Qixing Farm, Jiansanjiang, Northeast China from 2008 to 2018, and the dataset was used for the model calibration, validation, and evaluation. Two indirect and one direct NNI prediction methods using simple regression, stepwise multiple linear regression (SMLR) and random forest regression (RFR) were compared for N diagnosis and then integrated into N recommendation model. The results indicated that combining environmental and agronomic variables with crop sensor data improved the SMLR and RFR model performance by 1–16% and 9–40% over the corresponding models only using crop sensor data, respectively. The direct NNI prediction approach achieved slightly better N status diagnostic accuracy (areal agreement = 84% and Kappa statistics = 0.71) than indirect NNI prediction strategies based on plant N uptake and ΔN estimation (areal agreement = 81% and Kappa statistics = 0.67) or aboveground biomass and plant N uptake estimation (areal agreement = 77% and Kappa statistics = 0.58) across plot experiments and diverse on-farm conditions, based on multi-source data fusion with random forest regression models. About 82% of recommended N rates by the developed integrated in-season rice N diagnosis and recommendation model were within ±10 kg ha−1 of the measured economic optimum N rate across different varieties, environmental conditions and transplanting densities. Precision rice management based on in-season N diagnosis and recommendation decreased N rates and increased N partial factor productivity (PFPN) by 23% over regional optimum rice management, and significantly increased yield (7–11%) and PFPN (33–77%) over farmer's management. More studies are needed to develop in-season N diagnosis and recommendation strategies for applications across different regions and combine them with integrated precision rice management strategies for food security and sustainable development.
Abstract
This study investigated the potential of in-season airborne hyperspectral imaging for the calibration of robust forage yield and quality estimation models. An unmanned aerial vehicle (UAV) and a hyperspectral imager were used to capture canopy reflections of a grass-legume mixture in the range of 450 nm to 800 nm. Measurements were performed over two years at two locations in Southeast and Central Norway. All images were subject to radiometric and geometric corrections before being processed to ortho-images, carrying canopy reflectance information. The data (n = 707) was split in two, using half the data for model calibration and the remaining half for validation. Several powered partial least squares regression (PPLSR) models were fitted to the reflectance data to estimate fresh (FM) and dry matter (DM) yields, as well as crude protein (CP), dry matter digestibility (DMD), neutral detergent fibre (NDF), and indigestible neutral detergent fibre (iNDF) content. Prediction performance of these models was compared with the prediction performance of simple linear regression (SLR) models, which were based on selected vegetation indices and plant height. The highest prediction accuracies for general models, based on the pooled data, were achieved by means of PPLSR, with relative root-mean-square errors of validation of 14.2% (2550 kg FM ha−1), 15.2% (555 kg DM ha−1), 11.7% (1.32 g CP 100 g−1 DM), 2.4% (1.71 g DMD 100 g−1 DM), 4.8% (2.72 g NDF 100 g−1 DM), and 12.8% (1.32 g iNDF 100 g−1 DM) for the prediction of FM, DM, CP, DMD, NDF, and iNDF content, respectively. None of the tested SLR models achieved acceptable prediction accuracies.
Abstract
Hydroponic production of strawberry (Fragaria × ananassa Duch.) in protected cultivation systems using substrates (growing media) is gaining popularity worldwide. Therefore, it is necessary to develop more sustainable growing media alternatives. This study focused on growth performance of strawberry plants grown in wood fibre from Norway spruce (Picea abies (L.) H. Karst.), in comparison to two industry standards (peat and coco fibres). Plug (tray) plants of the June-bearing strawberry cultivar 'Malling Centenary' and bare root (WBH) plants of cultivar 'Sonata' were transplanted into three different growing media: peat (80%) and perlite (20%) mixture, coconut coir (100%) and Norway spruce wood fibre (100%). The plants received four fertigation strategies (various potassium and nitrogen concentrations) from flowering onwards. Throughout the production season ripe berries were harvested and frozen for later analyses of chemical composition. Plant architecture was also recorded after termination of the experiment. The results revealed that the most significant differences among the majority of the fruit and plant parameters were due to cultivar traits. Strawberries grown in wood fibre produced slightly smaller berries with elevated °Brix and dry matter compared to berries from plants grown in peat and coir. This was most likely caused by the common fertigation strategy applied to all substrates. Nevertheless, among the tested fertigation strategies, application of solutions with elevated potassium resulted in the highest sugar accumulation in berries grown in wood fibre substrate. In general, the experiment revealed relatively negligible differences between the growing media, and we therefore conclude that wood fibre from Norway spruce may be a viable alternative as a growing media in hydroponic strawberry production when the fertigation strategy is precisely adjusted.
Abstract
Høsten 2018 gjennomførte vi ved Senter for presisjonsjordbruk en test av spesialutviklet maskin (Veris MSP3) for «on-the-go» måling av pH, mold (organisk materiale; OM) og elektrisk ledeevne i jordbruksjord. Testen ble gjennomført på oppdrag av Franzefoss Minerals, som ønsket en objektiv evaluering av maskinen og dens egnethet under norske forhold. Maskinen ble testet i felt med spesielt fokus på pH målingene. Målinger av pH og OM som ble tatt med Veris ble sammenlignet med tilsvarende lab målinger fra jordprøver tatt fra de samme prøvestedene. Dataanalysen viste at det var svært dårlig samsvar mellom Veris- og lab målingene av både pH og OM. Maskinen har tidligere blitt testet både under kontrollerte, semi-kontrollerte og realistiske forhold i andre land, blant annet i USA og i Tyskland. Her har det blitt funnet en viss sammenheng mellom pH målinger av Veris og labmålinger. Grunnen til at Veris gjør det dårligere i vår test kan skyldes både maskinens tilstand, utforming og mangelfull evne til å håndtere stein. Denne rapporten redegjør detaljert for disse utfordringene.
Abstract
I denne utredningen har vi søkt å kartlegge omfanget av presisjonsjordbruk (PA) i Norge i dag og beregne potensiell miljømessig gevinst og kost-nytte-verdi ved å implementere slike metoder. PA blir ofte definert som en jordbrukspraksis der en bruker ny teknologi til å tilpasse behandlingen av jord og vekst etter behovet som ofte varierer mye innenfor samme jordet. Dette omfatter bl.a. variabel rate-teknologi (VRT), som innebærer at en kan fordele innsatsfaktorer som bl.a. kalk, gjødsel og plantevernmidler med varierende, stedsspesifikk dosering. PA utgjør dermed et alternativ til vanligste praksis i dag, der innsatsfaktorene tildeles jevnt over hele jordet (ensartet) ut fra gjennomsnittsbetraktninger.
Abstract
Det er ikke registrert sammendrag
Prosjekter
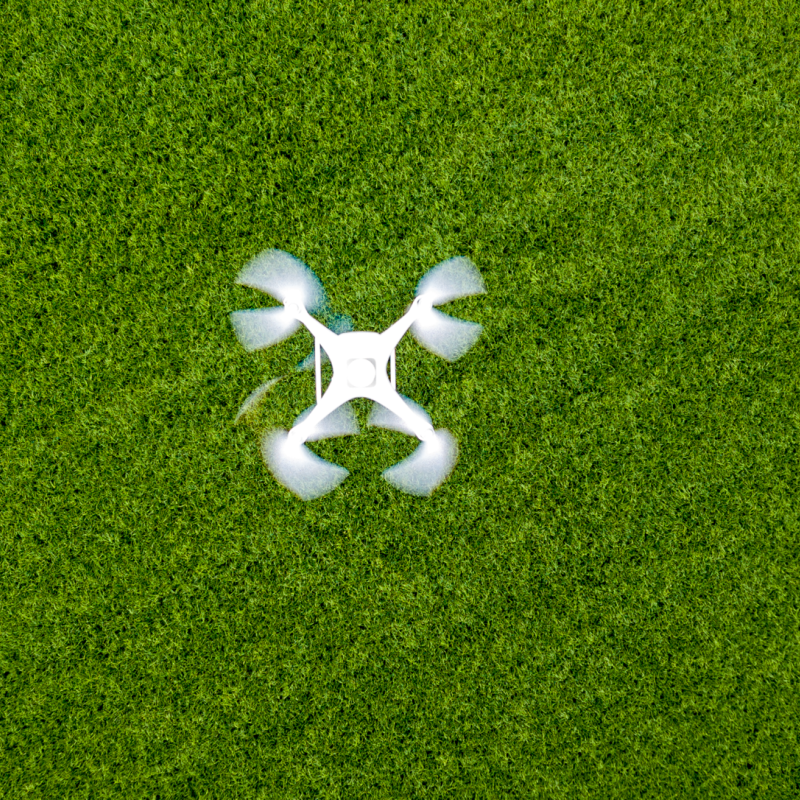
Divisjon for matproduksjon og samfunn
PRESIS
I PRESIS, et nytt femårig samarbeidsprosjekt, skal NIBIO være med å videreutvikle teknologiske løsninger for enda bedre presisjonsjordbruk, og samtidig sørge for at løsningene kommer ut i praksis til gårdene.
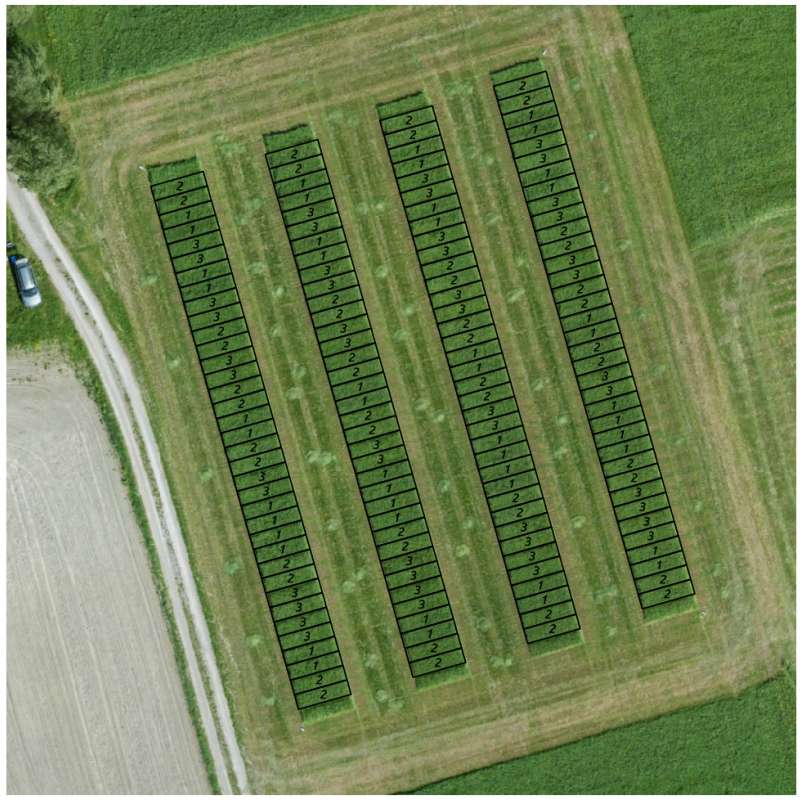
Divisjon for matproduksjon og samfunn
IMPRESS
IMPRESS - Økt presisjon og målretting i gjødsling og avlingsfremmende tiltak i grovfôrproduksjonen Hovedmålet med prosjektet er å heve avling og kvalitet i norsk grovfôrproduksjon gjennom å utvikle og tilpasse presisjonsteknologi, modeller og tiltak for stedsspesifikk bruk av innsatsfaktorer, og for identifikasjon og forbedring av lavtytende områder i enga
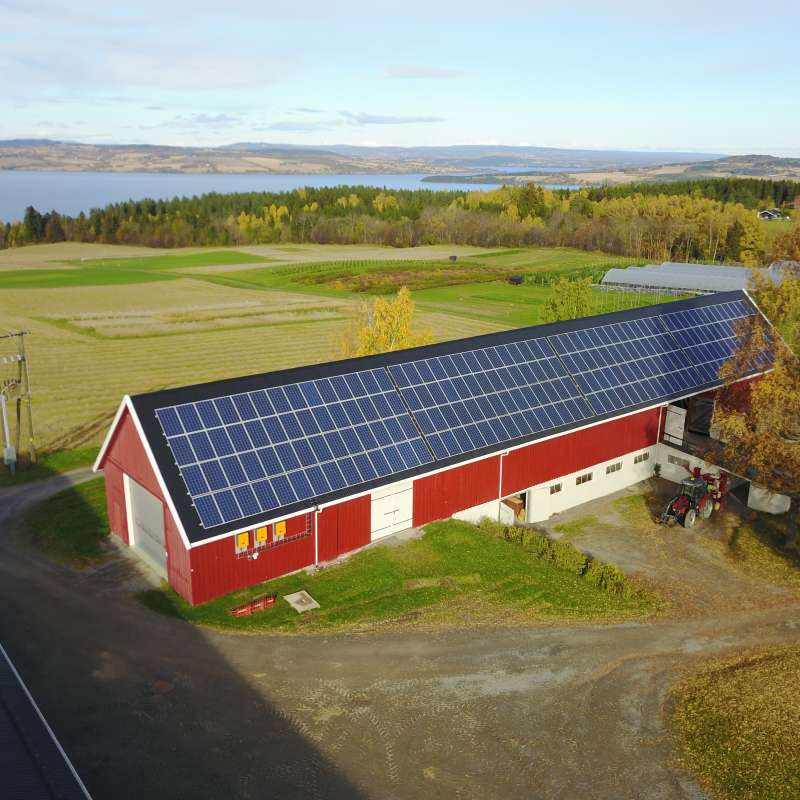
Divisjon for matproduksjon og samfunn
SolarFarm
SolarFarm - En systemstudie av hvordan solstrøm produsert på gårdsnivå kan drive elektriske og delvis selvstyrte farkoster i et presisjonsjordbruk med reduserte utslipp av klimagasser