Krzysztof Kusnierek
Avdelingsleder/forskningssjef
Sammendrag
Det er ikke registrert sammendrag
Sammendrag
Raman spectroscopy provides detailed information about the molecular composition of a sample. The classical identification of components in a multi-component sample typically involves comparing the preprocessed spectrum with a known reference stored in a database using various spectral matching or machine-learning techniques or relies on universal models based on a two-step analysis including first, the component identification, and then the decomposition of the mixed signal. However, although large databases and universal models cover a wide range of target materials, they may be not optimized to the variability required in a specific application. In this study, we propose a single-step method using deep learning (DL) modeling to decompose a simulated mixture of real measurements of Raman scattering into relevant individual components regardless of noise, baseline and the number of components involved and quantify their ratios. We hypothesize that training a custom DL model for applications with a fixed set of expected components may yield better results than applying a universal quantification model. To test this hypothesis, we simulated 12,000 Raman spectra by assigning random ratios to each component spectrum within a library containing 13 measured spectra of organic solvent samples. One of the DL methods, a fully connected network (FCN), was designed to work on the raw spectra directly and output the contribution of each component of the library to the input spectrum in form of a component ratio. The developed model was evaluated on 3600 testing spectra, which were simulated similarly to the training dataset. The average component identification accuracy of the FCN was 99.7%, which was significantly higher than that of the universal custom trained DeepRaman model, which was 83.1%. The average mean absolute error for component ratio quantification was 0.000562, over one order of magnitude smaller than that of a well-established non-negative elastic net (NN-EN), which was 0.00677. The predicted non-zero ratio values were further used for component identification. Under the assumption that the components of a mixture are from a fixed library, the proposed method preprocesses and decomposes the raw data in a single step, quantifying every component in a multicomponent mixture, accurately. Notably, the single-step FCN approach has not been implemented in the previously reported DL studies.
Sammendrag
Increasing planting densities and nitrogen (N) application rates are two practices commonly used in high-yield maize (Zea mays L.) production systems to increase crop yield, but have resulted in lower N use efficiency, increased lodging, and negative environmental problems. Crop sensing-based precision N management (PNM) strategies have been developed to optimize maize yield, N use efficiency, and reduce environmental footprints, however, PNM strategies to balance grain yield and lodging risks are still very limited. The objectives of this study were to: (1) propose a N nutrition index (NNI)-based algorithm for in-season estimation of maize N demand; and (2) develop a sensor-based PNM strategy to balance grain yield and lodging risk for maize. Field experiments were conducted in Northeast China from 2017 to 2019, using a split-plot design with three planting densities (5.5, 7.0 and 8.5 plants m−2) as main plots and six N rates (0–300 kg ha−1) as subplots. Based on previous studies, a leaf fluorescence sensor Dualex 4 good for estimating plant N concentration and a canopy reflectance sensor Crop Circle ACS 430 good for estimating plant aboveground biomass were used to estimate maize NNI and predict lodging risk. Total N rates to achieve low lodging risk were determined based on wind velocity causing maize stalk lodging and historical actual natural wind speed, as well as the response of a lodging risk indicator (stem failure moment, Bs) to N supply. In-season side-dress N rates were determined based on theoretical amount of preplant N fertilizer estimated using NNI and a target total N rate. The final recommended sidedress N rates were adjusted based on the sensor-predicted lodging risk. The results indicated that NNI could be used for estimating the theoretical amount of preplant N fertilizer required to reach the current N status. It’s feasible to estimate maize side-dress N demand based on the difference of a target total N rate (to achieve an optimal grain yield or low lodging risk) and the current theoretical N supply. Total N rate to ensure low lodging risk was suggested to be adopted under low and medium planting densities. Medium planting density of 70,000 plants ha−1 matched with the corresponding optimal N rate would be recommended for the study area to balance economic return and lodging risk. In general, high planting density is not recommended because it has high lodging risk. More studies are needed to further improve the developed crop sensing-based PNM strategy with more site-years of data and multi-source data fusion using machine learning models for practical on-farm applications.
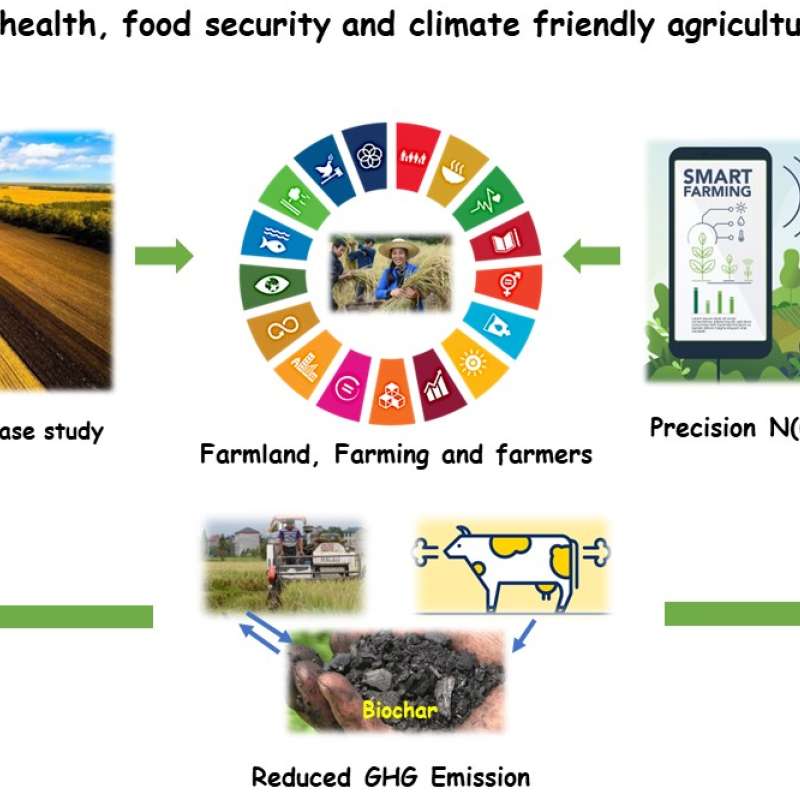
Divisjon for miljø og naturressurser
Sinograin III: Smart agricultural technology and waste-made biochar for food security, reduction of greenhouse gas (GHG) emission, and bio-and circular economy
The Sinograin III project’s overall objective is to contribute to the UN SDGs by widely implementing precision agriculture technologies and application of “waste-to-value” biochar products to achieve sustainable food production with minimized GHG emission, improve soil fertility and promote green growth/zero waste in modern agriculture in China.
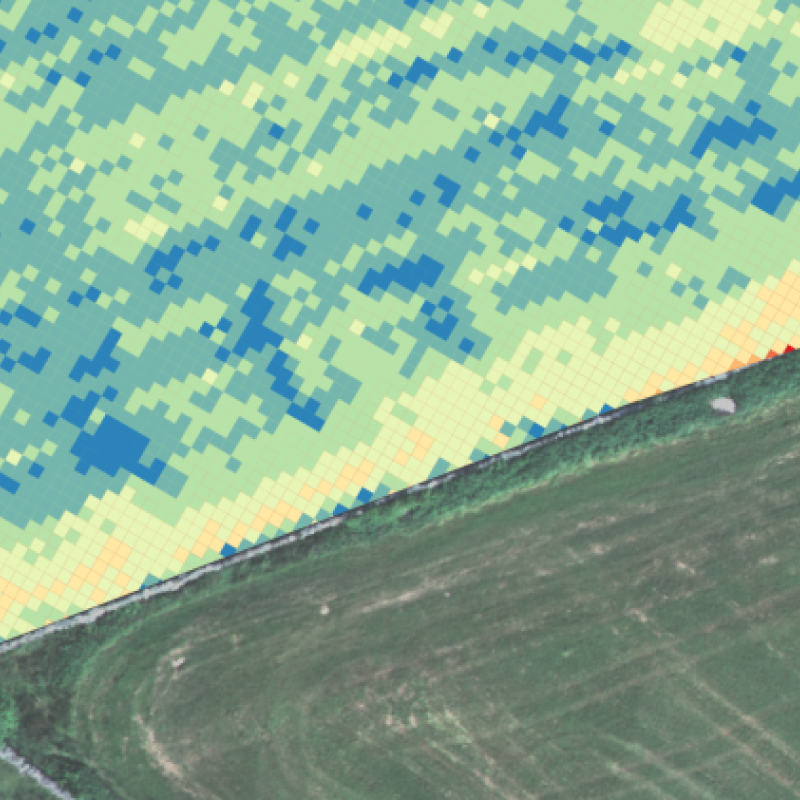
Divisjon for matproduksjon og samfunn
COPERNICUS - Jordbruk
Formålet med prosjektet er å ta i bruk satellitt-data fra Copernicus programmet for å utvikle rutiner og verktøy rettet inn mot jordbruksfaglige problemstillinger, og gjennom dette bidra med informasjon og råd til relevante aktører (bønder, rådgivere, jordbruksnæringa, kommuner, politikere og utdanningsinstitusjoner). Prosjektet skal dermed bidra til å forbedre dagens dyrkningspraksis, som gjennom en bedre utnyttelse av innsatsfaktorer som gjødsel og fôr også bidrar til å redusere klimaavtrykket til det norske jordbruket.
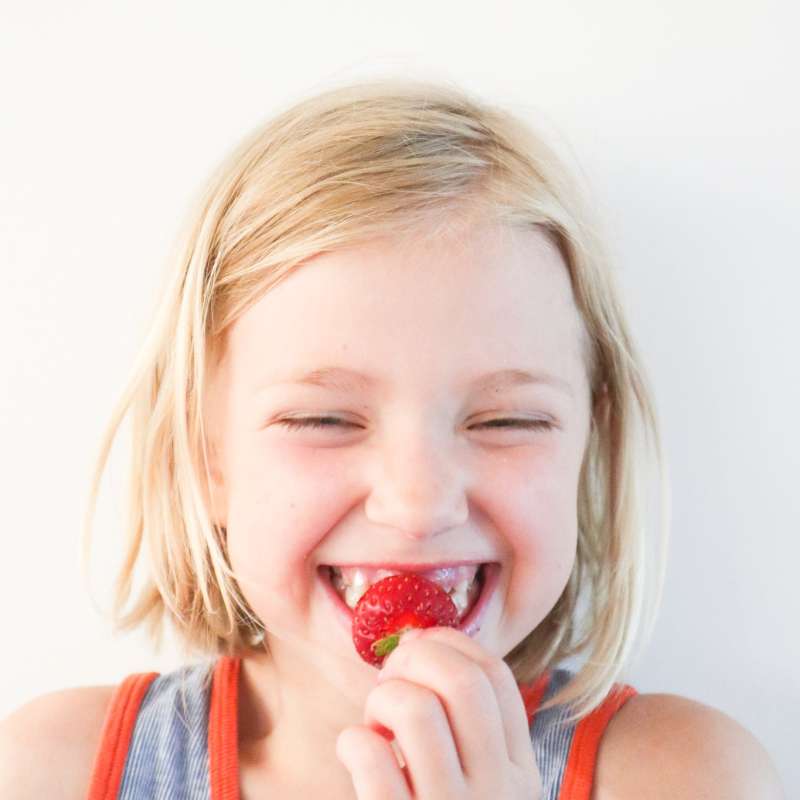
Divisjon for matproduksjon og samfunn
JordbærSmak: En optimalisert moderne produksjonsteknologi for mer smakfulle norske jordbær
Det er et mål å øke produksjonen i den norske grøntsektoren, inkludert jordbær, med inntil 50 prosent de kommende 15 årene. For å oppnå dette må dyrkingssesongen utvides, men da trengs en mye bedre kunnskap om hvordan man kan påvirke planteveksten og ta i bruk teknologi for å overvåke og beskytte plantene, uten at det går utover kvalitet og smak.
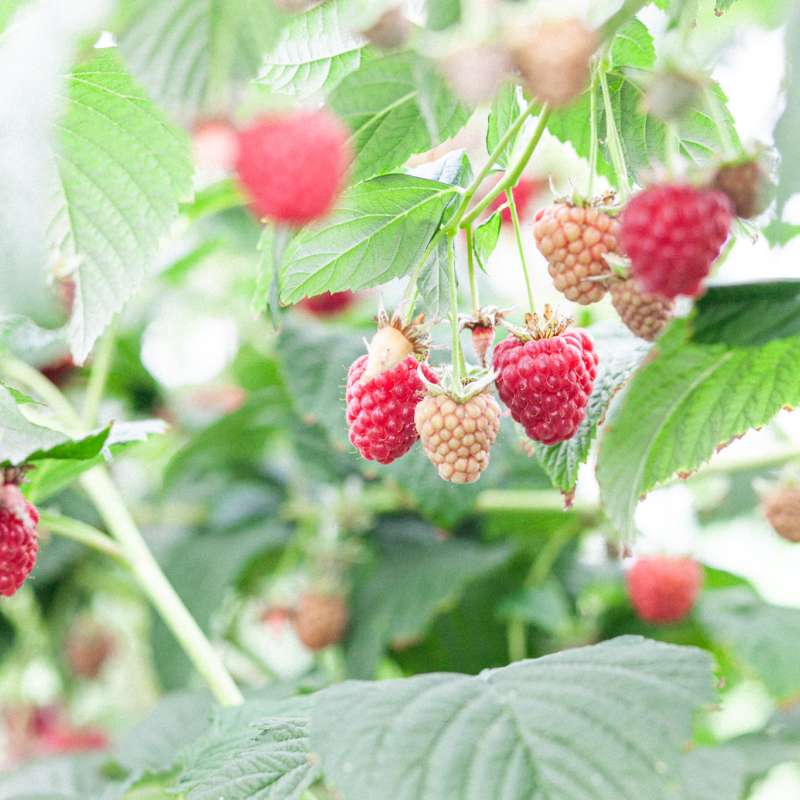
Divisjon for matproduksjon og samfunn
RobustRubus - En optimalisert og klimatilpassa produksjon av bringebær og bjørnebær i en forlenget sesong
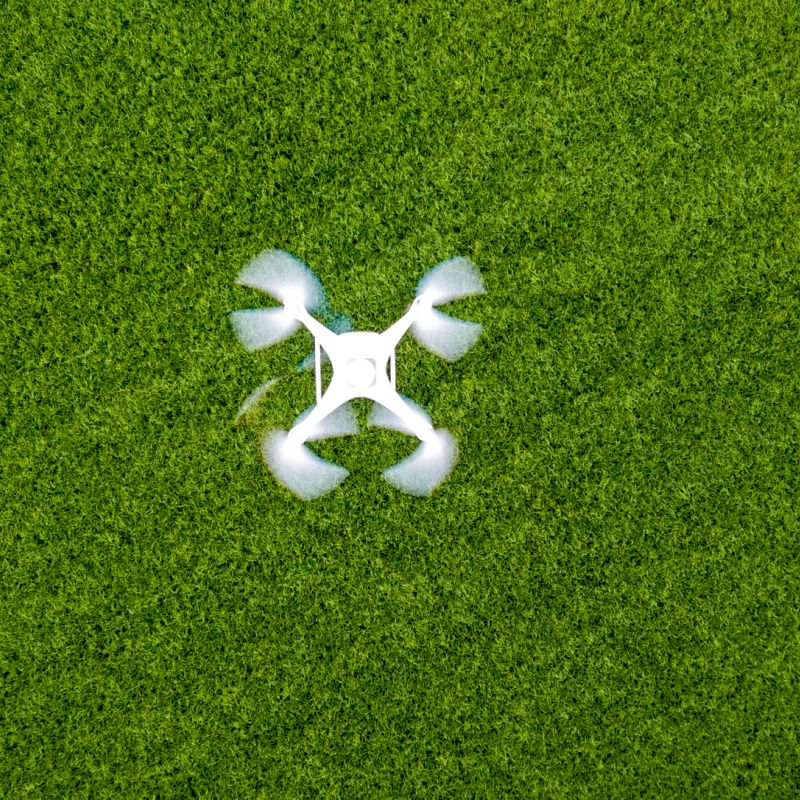
Divisjon for matproduksjon og samfunn
PRESIS
I PRESIS, et nytt femårig samarbeidsprosjekt, skal NIBIO være med å videreutvikle teknologiske løsninger for enda bedre presisjonsjordbruk, og samtidig sørge for at løsningene kommer ut i praksis til gårdene.
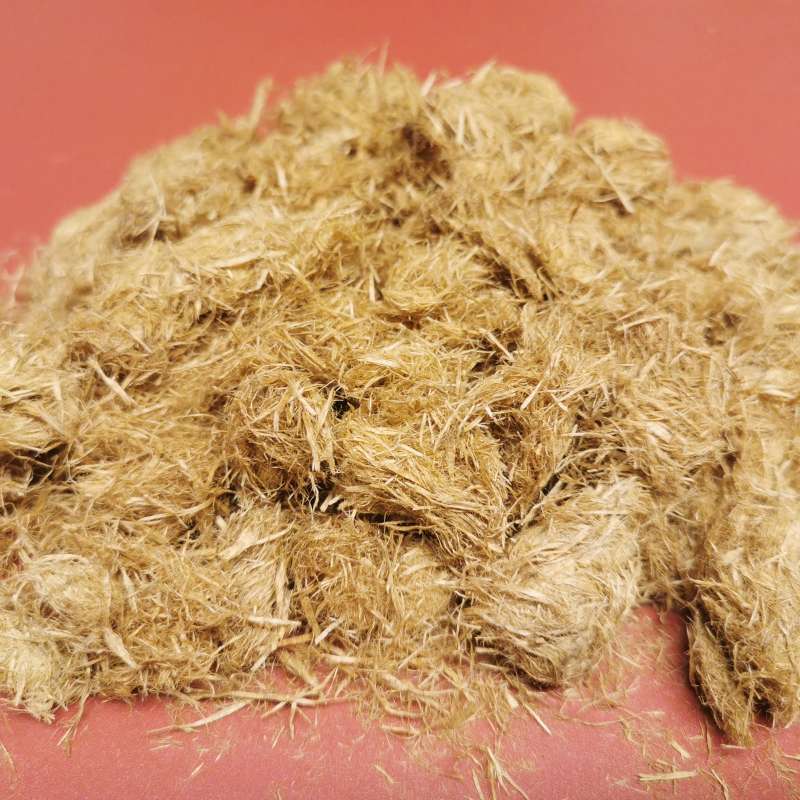
Divisjon for matproduksjon og samfunn
Utnyttelse av trefiber og avfallsstrømmer for bærekraftige og sirkulære dyrkingsmedier i hagebruket (‘SUBTECH 2.0’)
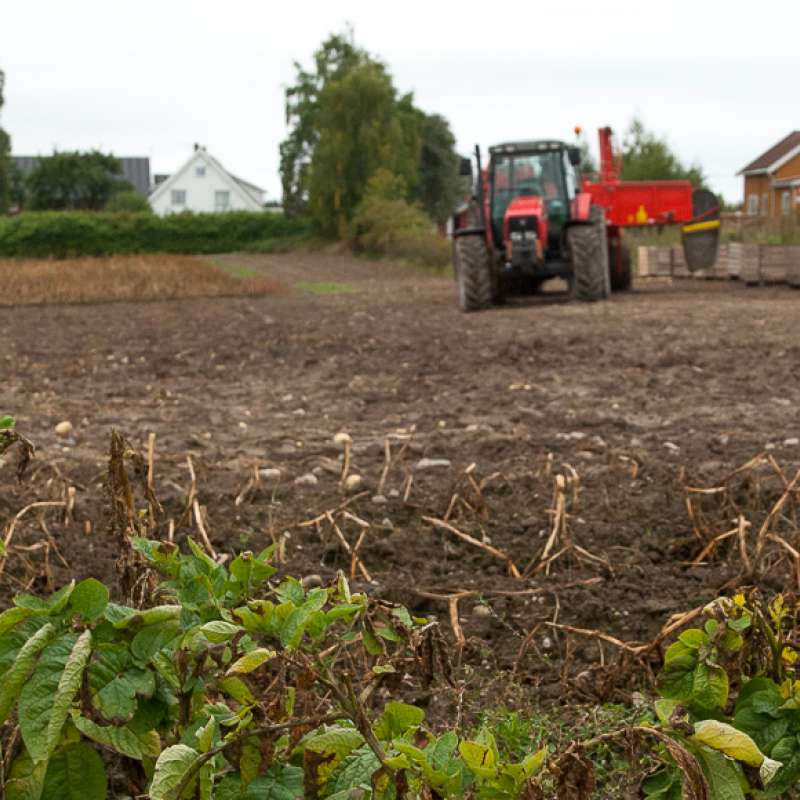
Divisjon for bioteknologi og plantehelse
SOLUTIONS: Nye løsninger for nedvisning av potetris, bekjempelse av ugras og utløpere i jordbær og ugraskontroll i eplehager
Håndtering av ugress og andre plantevernutfordringer er viktig for å unngå avlingstap i landbruket. Tilbudet av norske rå-, mat- og fôrvarer påvirkes av at bonden lykkes med sin innsats i åker og frukthager. Et nylig forbud mot plantevernmiddelet dikvat og den usikre framtida til glyfosat – begge viktige innsatsfaktorer i norsk jord- og hagebruk – fordrer nye løsninger. Gode alternativ til ordinære plantevernmidler er dessuten velkomne som verktøy i integrert plantevern (IPV). Norske dyrkere er siden 2015 pålagt å følge IPV. Hensikten med IPV er blant annet redusert risiko ved bruk av plantevernmidler på helse og miljø.