Jakob Geipel
Forsker
Biografi
Jakob er en forsker som er ekspert på sensorer og bruk av satellitter, droner, traktorer og roboter til datafangst i jordbruket. Han jobber bl.a. med multi- og hyperspektral fjernanalyse, bildebehandling og modellering av plantegenskaper med fokus på avlings- og kvalitetsestimering for presisjonsjordbruk. Hans forskningsaktivitet omfatter både fôr-, korn og potetproduksjon.
Forfattere
Therese With Berge Jiangsan Zhao Jakob Geipel El Houssein Chouaib Harik Malin Larsen Græsdahl Marit HelgheimSammendrag
Det er ikke registrert sammendrag
Forfattere
Jakob GeipelSammendrag
Det er ikke registrert sammendrag
Forfattere
Jakob GeipelSammendrag
Det er ikke registrert sammendrag
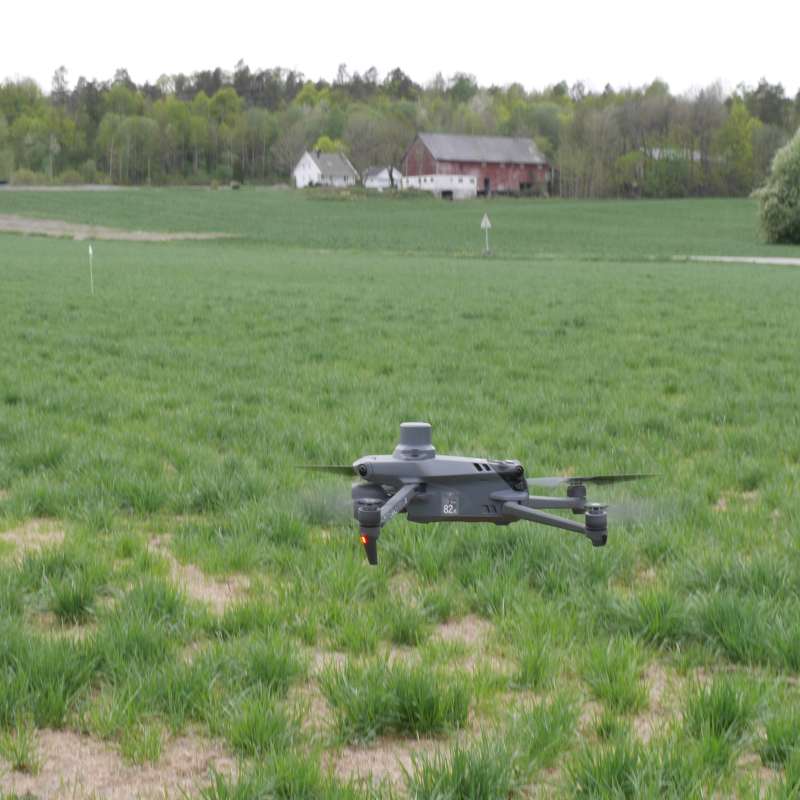
Divisjon for matproduksjon og samfunn
Presis gjødsling og vekstregulering av norske grasfrøenger ved hjelp av sensorteknologi (SmartSeed)
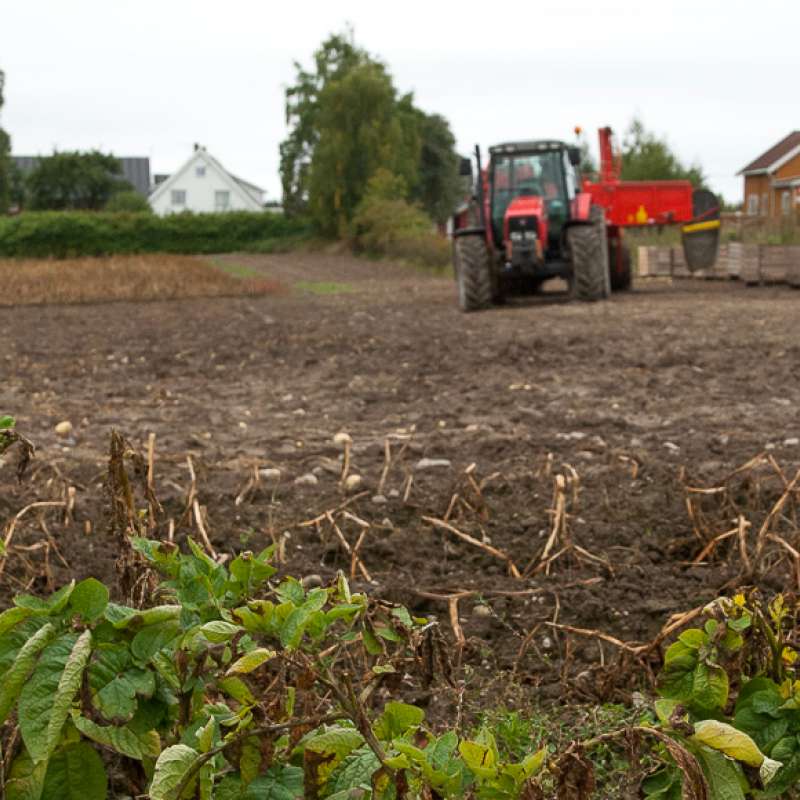
Divisjon for bioteknologi og plantehelse
SOLUTIONS: New solutions for potato canopy desiccation, control of weeds and runners in field strawberries & weed control in apple orchards
Efficient measures for weed control and similar challenges are vital to avoid crop loss in agriculture. National supply of food, feed and other agricultural products depends on each farmer’s success managing their fields and orchards. The recent loss of the herbicide diquat, and the potential ban on glyphosate, - both important tools for farmers -, raise a demand for new measures for vegetation control. Efficient alternatives to herbicides are also important tools in Integrated Pest Management (IPM). Norwegian growers need to document compliance to IPM since 2015 to ensure minimum hazards to health and environment from pesticide use.
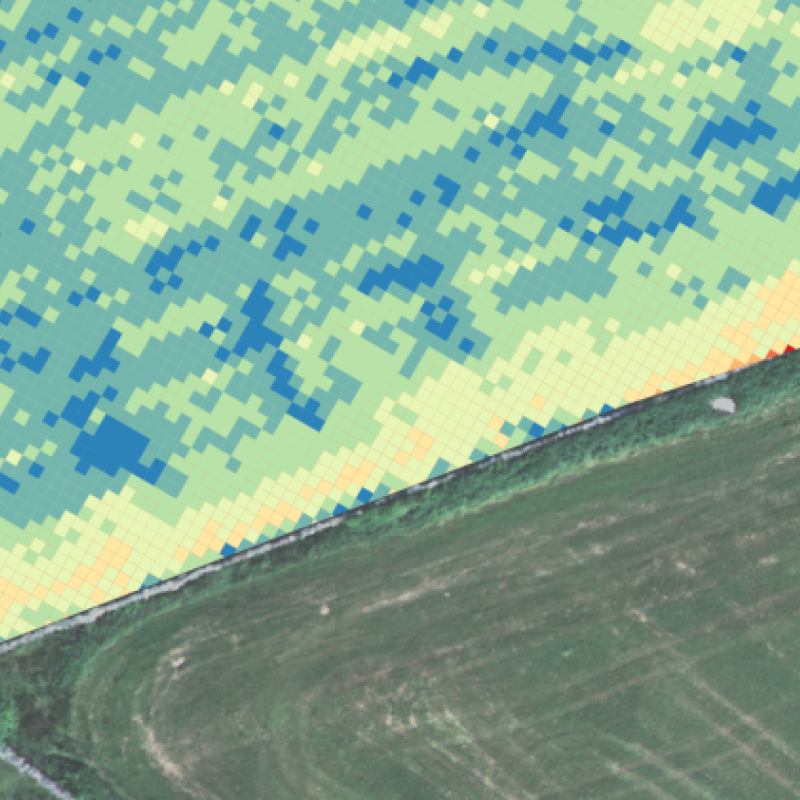
Divisjon for matproduksjon og samfunn
COPERNICUS - Jordbruk
Formålet med prosjektet er å ta i bruk satellitt-data fra Copernicus programmet for å utvikle rutiner og verktøy rettet inn mot jordbruksfaglige problemstillinger, og gjennom dette bidra med informasjon og råd til relevante aktører (bønder, rådgivere, jordbruksnæringa, kommuner, politikere og utdanningsinstitusjoner). Prosjektet skal dermed bidra til å forbedre dagens dyrkningspraksis, som gjennom en bedre utnyttelse av innsatsfaktorer som gjødsel og fôr også bidrar til å redusere klimaavtrykket til det norske jordbruket.
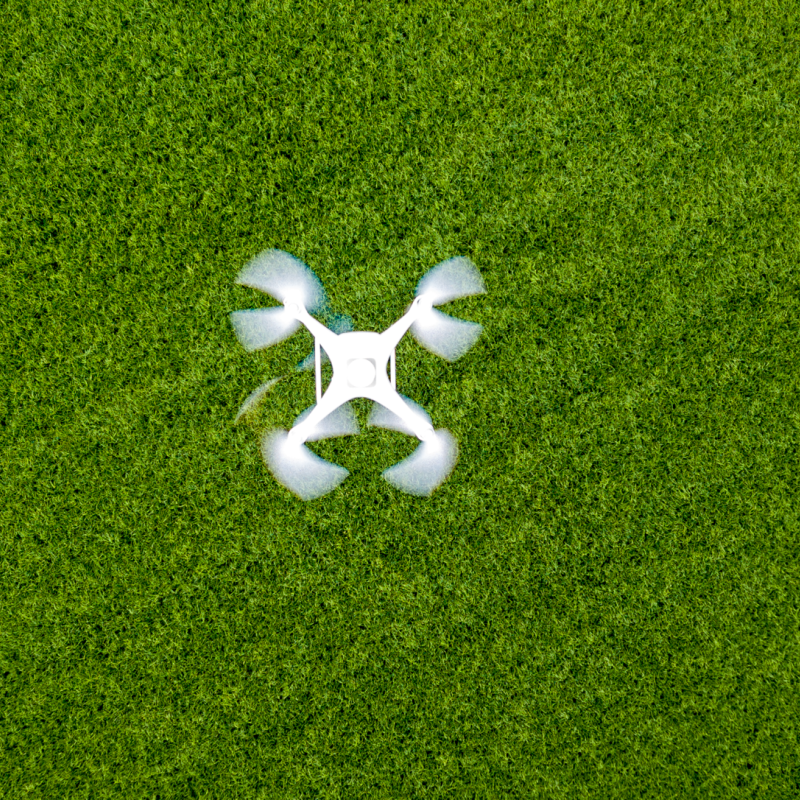
Divisjon for matproduksjon og samfunn
PRESIS
I PRESIS, et nytt femårig samarbeidsprosjekt, skal NIBIO være med å videreutvikle teknologiske løsninger for enda bedre presisjonsjordbruk, og samtidig sørge for at løsningene kommer ut i praksis til gårdene.
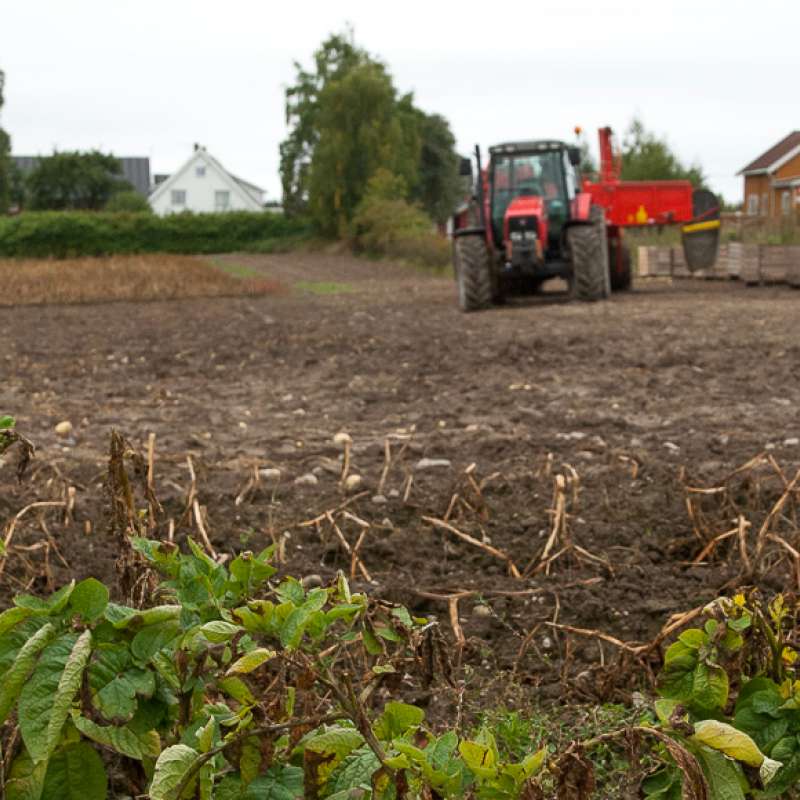
Divisjon for bioteknologi og plantehelse
SOLUTIONS: Nye løsninger for nedvisning av potetris, bekjempelse av ugras og utløpere i jordbær og ugraskontroll i eplehager
Håndtering av ugress og andre plantevernutfordringer er viktig for å unngå avlingstap i landbruket. Tilbudet av norske rå-, mat- og fôrvarer påvirkes av at bonden lykkes med sin innsats i åker og frukthager. Et nylig forbud mot plantevernmiddelet dikvat og den usikre framtida til glyfosat – begge viktige innsatsfaktorer i norsk jord- og hagebruk – fordrer nye løsninger. Gode alternativ til ordinære plantevernmidler er dessuten velkomne som verktøy i integrert plantevern (IPV). Norske dyrkere er siden 2015 pålagt å følge IPV. Hensikten med IPV er blant annet redusert risiko ved bruk av plantevernmidler på helse og miljø.