Stefano Puliti
Forsker
Sammendrag
RoadSens is a platform designed to expedite the digitalization process of forest roads, a cornerstone of efficient forest operations and management. We incorporate stereo-vision spatial mapping and deep-learning image segmentation to extract, measure, and analyze various geometric features of the roads. The features are precisely georeferenced by fusing post-processing results of an integrated global navigation satellite system (GNSS) module and odometric localization data obtained from the stereo camera. The first version of RoadSens, RSv1, provides measurements of longitudinal slope, horizontal/vertical radius of curvature and various cross-sectional parameters, e.g., visible road width, centerline/midpoint positions, left and right sidefall slopes, and the depth and distance of visible ditches from the road’s edges. The potential of RSv1 is demonstrated and validated through its application to two road segments in southern Norway. The results highlight a promising performance. The trained image segmentation model detects the road surface with the precision and recall values of 96.8 and 81.9 , respectively. The measurements of visible road width indicate sub-decimeter level inter-consistency and 0.38 m median accuracy. The cross-section profiles over the road surface show 0.87 correlation and 9.8 cm root mean squared error (RMSE) against ground truth. The RSv1’s georeferenced road midpoints exhibit an overall accuracy of 21.6 cm in horizontal direction. The GNSS height measurements, which are used to derive longitudinal slope and vertical curvature exhibit an average error of 5.7 cm compared to ground truth. The study also identifies and discusses the limitations and issues of RSv1, which provide useful insights into the challenges in future versions.
Sammendrag
Det er ikke registrert sammendrag
Forfattere
Binbin Xiang Maciej Wielgosz Theodora Kontogianni Torben Peters Stefano Puliti Rasmus Astrup Konrad SchindlerSammendrag
Detailed forest inventories are critical for sustainable and flexible management of forest resources, to conserve various ecosystem services. Modern airborne laser scanners deliver high-density point clouds with great potential for fine-scale forest inventory and analysis, but automatically partitioning those point clouds into meaningful entities like individual trees or tree components remains a challenge. The present study aims to fill this gap and introduces a deep learning framework, termed ForAINet, that is able to perform such a segmentation across diverse forest types and geographic regions. From the segmented data, we then derive relevant biophysical parameters of individual trees as well as stands. The system has been tested on FOR-Instance, a dataset of point clouds that have been acquired in five different countries using surveying drones. The segmentation back-end achieves over 85% F-score for individual trees, respectively over 73% mean IoU across five semantic categories: ground, low vegetation, stems, live branches and dead branches. Building on the segmentation results our pipeline then densely calculates biophysical features of each individual tree (height, crown diameter, crown volume, DBH, and location) and properties per stand (digital terrain model and stand density). Especially crown-related features are in most cases retrieved with high accuracy, whereas the estimates for DBH and location are less reliable, due to the airborne scanning setup.
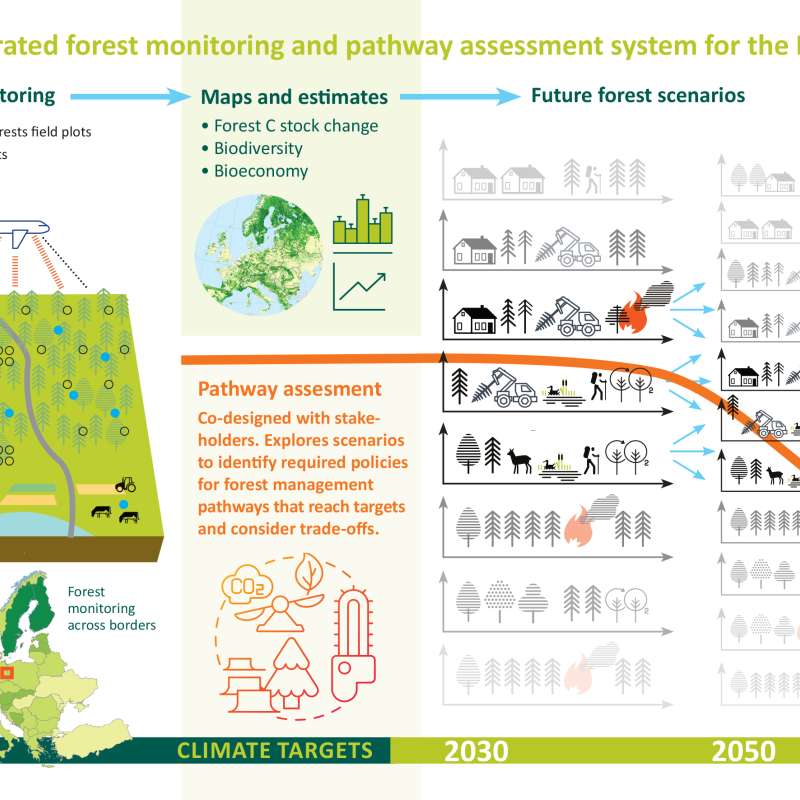
Divisjon for skog og utmark
PathFinder
Towards an Integrated Consistent European LULUCF Monitoring and Policy Pathway Assessment Framework

Divisjon for skog og utmark
Lukkede hogster: Konsekvenser for produksjon, økonomi og biomangfold
Hovedmålet til prosjektet er å bygge kunnskap og kompetanse om hvordan en omlegging av norsk skogbruk, fra flatehogst til mer bruk av lukkede hogster, vil påvirke produksjon, økonomi og biologisk mangfold.
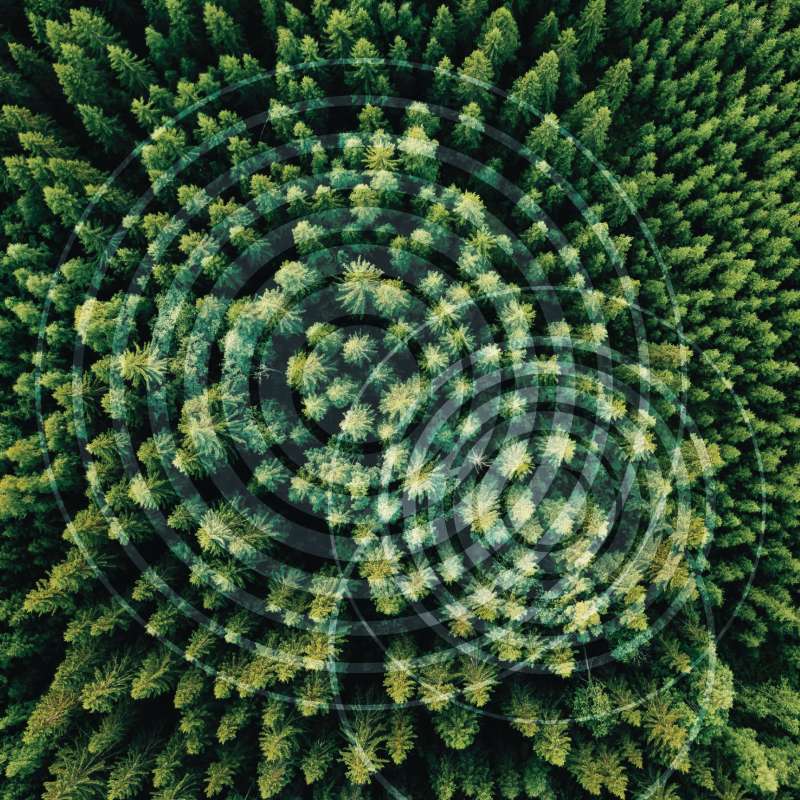
Divisjon for skog og utmark
SFI SmartForest: Bringing Industry 4.0 to the Norwegian forest sector
SmartForest will position the Norwegian forest sector at the forefront of digitalization resulting in large efficiency gains in the forest sector, increased production, reduced environmental impacts, and significant climate benefits. SmartForest will result in a series of innovations and be the catalyst for an internationally competitive forest-tech sector in Norway. The fundamental components for achieving this are in place; a unified and committed forest sector, a leading R&D environment, and a series of progressive data and technology companies.