Johannes Breidenbach
Avdelingsleder/forskningssjef
(+47) 974 77 985
johannes.breidenbach@nibio.no
Sted
Ås - Bygg H8
Besøksadresse
Høgskoleveien 8, 1433 Ås
Forfattere
Lennart Noordermeer Terje Gobakken Johannes Breidenbach Rune Eriksen Erik Næsset Hans Ole Ørka Ole Martin BollandsåsSammendrag
Det er ikke registrert sammendrag
Forfattere
Daniel Moreno-Fernández Johannes Breidenbach Isabel Cañellas Gherardo Chirici Giovanni D’amico Marco Ferretti Francesca Giannetti Stefano Puliti Sebastian Schnell Ross Shackleton Mitja Skudnik Iciar AlberdiSammendrag
Det er ikke registrert sammendrag
Sammendrag
På 17- og 1800-tallet var dimensjons- og plukkhogster de dominerende hogstformene i Norge. Resultatet var store områder med glissent bestokket skog og redusert produksjon. Etter andre verdenskrig ble bestandsskogbruk dominerende, med snauhogst og etterfølgende planting som den vanligst forekommende metode for høsting av skogressursene. Flatehogstene har påvirket store skogarealer og mengden naturskog vi har i dag. Dette har ført til debatt om omfanget av flatehogst og medførende påvirkning på skogstruktur og naturmangfold. Målet med arbeidet har vært å estimere hvor stor del av skogarealet i Norge som har vært flatehogd etter 1940 for å støtte debatten med et bedre tallgrunnlag. Resultatet er basert på data fra Landsskogtakseringen og tolkning av flybilder. Fylkene på Østlandet ble prioritert i første del av arbeidet, og vi presenterer her statistikk over hvor mye av skogen som er flatehogd i denne landsdelen, basert på at vi ved utvalgskartlegging har kunnet fastslå om skogen har vært gjenstand for flatehogst eller ikke på 95 % av skogarealet på Østlandet. 44 – 49 % av dagens skogareal på Østlandet har vært flatehogd etter 1940. Den uproduktive skogen er lite berørt (2 – 6 %), mens for den produktive skogen er andel flatehogd areal 53 – 58 %. Andel flatehogd areal er sterkt koblet til bonitet, og for gran- og furudominert skog på høy bonitet er henholdsvis 85 – 90 % og 83 – 92 % flatehogd, mens på lav bonitet er 21 – 25 % flatehogd. Skog i lavereliggende strøk har vært mer påvirket av flatehogst enn skog i høyereliggende strøk. Mellom 65 og 70 % av den produktive skogen under 600 moh. er flatehogd. For høyereliggende produktiv skog (> 800 moh.) er andelen vesentlig lavere, 14 – 22 %. Dette gjenspeiler også at skog med høyere bonitet er mer vanlig i lavtliggende strøk og er lettere tilgjengelig.
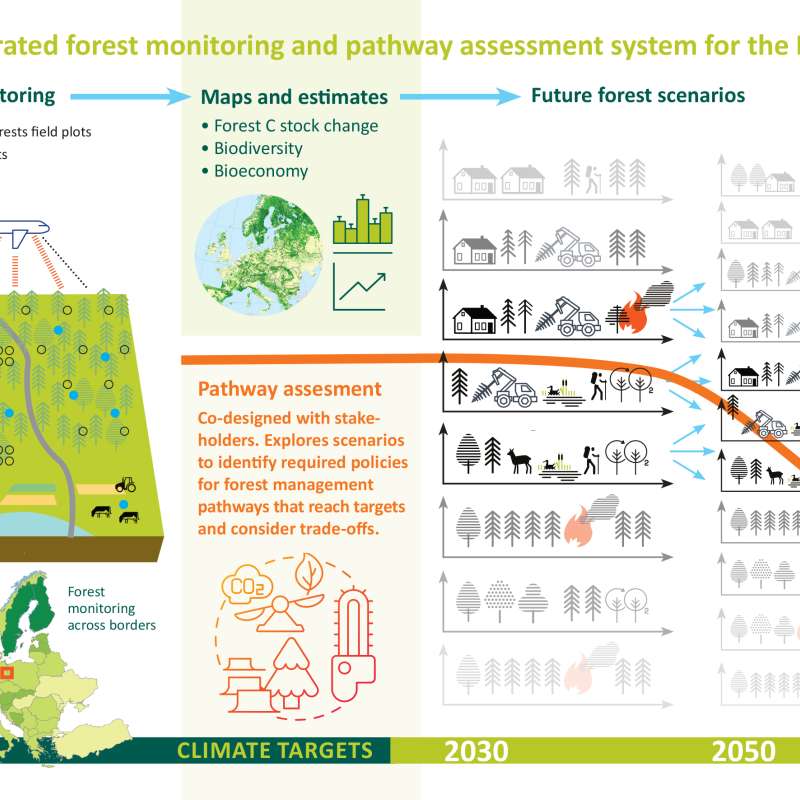
Divisjon for skog og utmark
PathFinder
Towards an Integrated Consistent European LULUCF Monitoring and Policy Pathway Assessment Framework
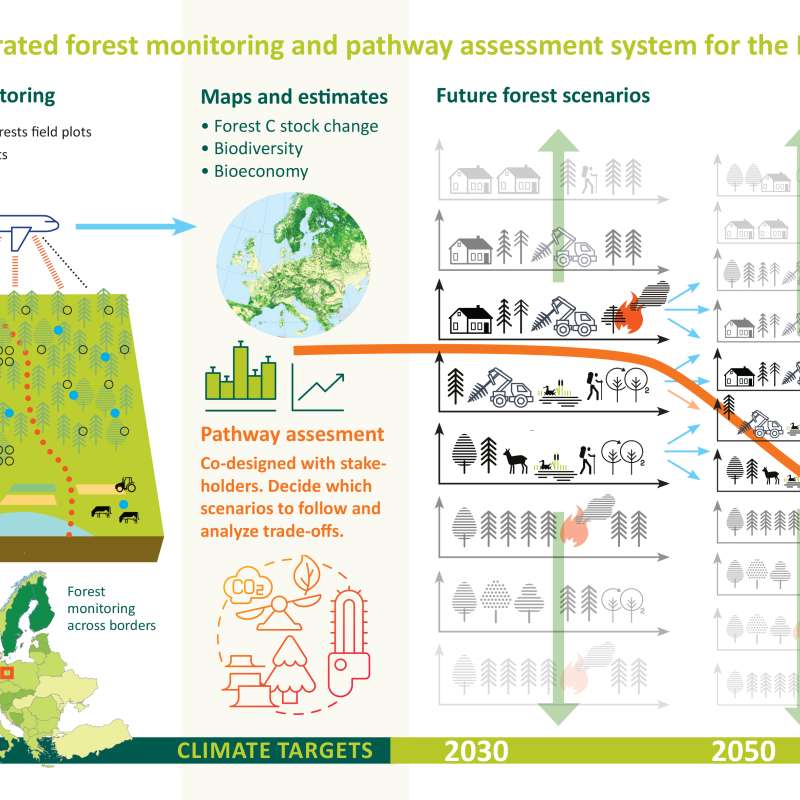
Divisjon for skog og utmark
PathFinder
Towards an Integrated Consistent European LULUCF Monitoring and Policy Pathway Assessment Framework
Se prosjektets offisielle nettside for mer informasjon:
https://pathfinder-heu.eu/
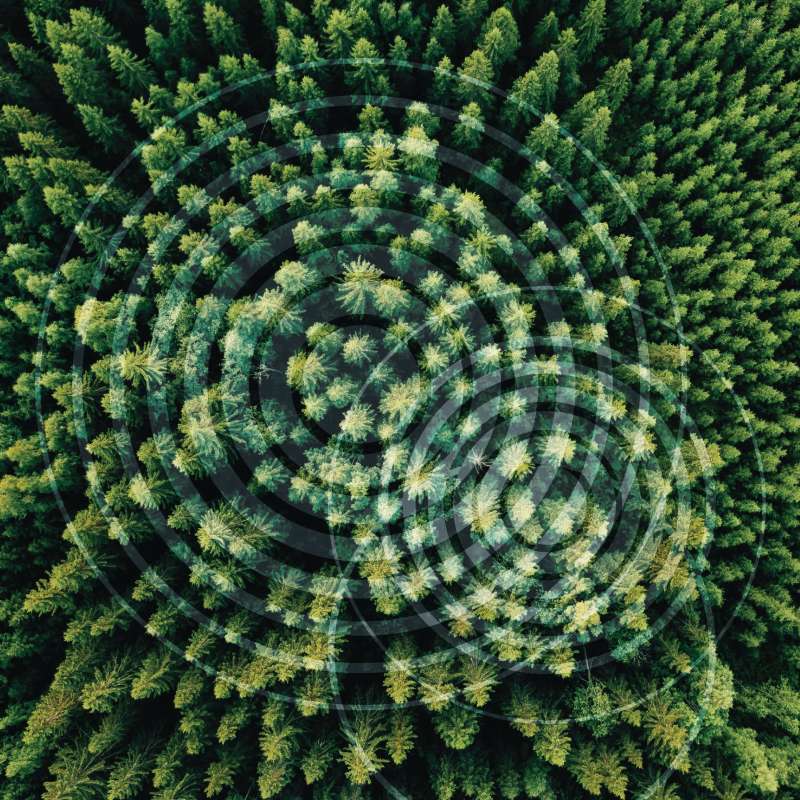
Divisjon for skog og utmark
SFI SmartForest: Bringing Industry 4.0 to the Norwegian forest sector
SmartForest will position the Norwegian forest sector at the forefront of digitalization resulting in large efficiency gains in the forest sector, increased production, reduced environmental impacts, and significant climate benefits. SmartForest will result in a series of innovations and be the catalyst for an internationally competitive forest-tech sector in Norway. The fundamental components for achieving this are in place; a unified and committed forest sector, a leading R&D environment, and a series of progressive data and technology companies.