Krzysztof Kusnierek
Head of Department/Head of Research
(+47) 920 12 953
krzysztof.kusnierek@nibio.no
Place
Apelsvoll
Visiting address
Nylinna 226, 2849 Kapp
Abstract
Raman spectroscopy provides detailed information about the molecular composition of a sample. The classical identification of components in a multi-component sample typically involves comparing the preprocessed spectrum with a known reference stored in a database using various spectral matching or machine-learning techniques or relies on universal models based on a two-step analysis including first, the component identification, and then the decomposition of the mixed signal. However, although large databases and universal models cover a wide range of target materials, they may be not optimized to the variability required in a specific application. In this study, we propose a single-step method using deep learning (DL) modeling to decompose a simulated mixture of real measurements of Raman scattering into relevant individual components regardless of noise, baseline and the number of components involved and quantify their ratios. We hypothesize that training a custom DL model for applications with a fixed set of expected components may yield better results than applying a universal quantification model. To test this hypothesis, we simulated 12,000 Raman spectra by assigning random ratios to each component spectrum within a library containing 13 measured spectra of organic solvent samples. One of the DL methods, a fully connected network (FCN), was designed to work on the raw spectra directly and output the contribution of each component of the library to the input spectrum in form of a component ratio. The developed model was evaluated on 3600 testing spectra, which were simulated similarly to the training dataset. The average component identification accuracy of the FCN was 99.7%, which was significantly higher than that of the universal custom trained DeepRaman model, which was 83.1%. The average mean absolute error for component ratio quantification was 0.000562, over one order of magnitude smaller than that of a well-established non-negative elastic net (NN-EN), which was 0.00677. The predicted non-zero ratio values were further used for component identification. Under the assumption that the components of a mixture are from a fixed library, the proposed method preprocesses and decomposes the raw data in a single step, quantifying every component in a multicomponent mixture, accurately. Notably, the single-step FCN approach has not been implemented in the previously reported DL studies.
Abstract
Increasing planting densities and nitrogen (N) application rates are two practices commonly used in high-yield maize (Zea mays L.) production systems to increase crop yield, but have resulted in lower N use efficiency, increased lodging, and negative environmental problems. Crop sensing-based precision N management (PNM) strategies have been developed to optimize maize yield, N use efficiency, and reduce environmental footprints, however, PNM strategies to balance grain yield and lodging risks are still very limited. The objectives of this study were to: (1) propose a N nutrition index (NNI)-based algorithm for in-season estimation of maize N demand; and (2) develop a sensor-based PNM strategy to balance grain yield and lodging risk for maize. Field experiments were conducted in Northeast China from 2017 to 2019, using a split-plot design with three planting densities (5.5, 7.0 and 8.5 plants m−2) as main plots and six N rates (0–300 kg ha−1) as subplots. Based on previous studies, a leaf fluorescence sensor Dualex 4 good for estimating plant N concentration and a canopy reflectance sensor Crop Circle ACS 430 good for estimating plant aboveground biomass were used to estimate maize NNI and predict lodging risk. Total N rates to achieve low lodging risk were determined based on wind velocity causing maize stalk lodging and historical actual natural wind speed, as well as the response of a lodging risk indicator (stem failure moment, Bs) to N supply. In-season side-dress N rates were determined based on theoretical amount of preplant N fertilizer estimated using NNI and a target total N rate. The final recommended sidedress N rates were adjusted based on the sensor-predicted lodging risk. The results indicated that NNI could be used for estimating the theoretical amount of preplant N fertilizer required to reach the current N status. It’s feasible to estimate maize side-dress N demand based on the difference of a target total N rate (to achieve an optimal grain yield or low lodging risk) and the current theoretical N supply. Total N rate to ensure low lodging risk was suggested to be adopted under low and medium planting densities. Medium planting density of 70,000 plants ha−1 matched with the corresponding optimal N rate would be recommended for the study area to balance economic return and lodging risk. In general, high planting density is not recommended because it has high lodging risk. More studies are needed to further improve the developed crop sensing-based PNM strategy with more site-years of data and multi-source data fusion using machine learning models for practical on-farm applications.
Abstract
Context Traditional critical nitrogen (N) dilution curve (CNDC) construction for N nutrition index (NNI) determination has limitations for in-season crop N diagnosis and recommendation under diverse on-farm conditions. Objectives This study was conducted to (i) develop a new rice (Oryza sativa L.) critical N concentration (Nc) determination approach using vegetation index-based CNDCs; and (ii) develop an N recommendation strategy with this new Nc determination approach and evaluate its reliability and practicality. Methods Five years of plot and on-farm experiments involving three japonica rice varieties were conducted at fourteen sites in Qixing Farm, Northeast China. Two machine learning (ML) methods, random forest (RF) and extended gradient boosting (XGBoost) regression, were used to fuse multi-source data including genotype, environment, management, growth stage, normalized difference vegetation index (NDVI) and normalized difference red edge (NDRE) from portable active canopy sensor RapidSCAN. The CNDC was established using NDVI and NDRE instead of aboveground biomass (AGB) measured by destructive sampling. A new in-season N diagnosis and recommendation strategy was further developed using direct and indirect NNI prediction using multi-source data fusion and ML models. Results The new CNDC based on NDVI or NDRE explained 94−96 % of Nc variability in the evaluation dataset when it was coupled with environmental and agronomic factors using ML models. The ML-based PNC and NNI prediction models explained 85 % and 21–36 % more variability over simple regression models using NDVI or NDRE in the evaluation dataset, respectively. The new in-season N diagnosis strategy using the NDVI and NDRE-based CNDCs and plant N concentration (PNC) predicted with RF model and multi-source data fusion performed slightly better than direct NNI prediction, explaining 7 % more of NNI variability and achieving 89 % of the areal agreement for N diagnosis across all evaluation experiments. Integrating this new N management strategy into the precision rice management system (as ML_PRM) increased yield, N use efficiency (NUE) and economic benefits over farmer’s practice (FP) by 7–15 %, 11–71 % and 4–16 % (161–596 $ ha−1), respectively, and increased NUE by 11–26 % and economic benefits by 8–97 $ ha−1 than regional optimum rice management (RORM) under rice N surplus status under on-farm conditions. Conclusions In-season rice N status diagnosis can be improved using NDVI- and NDRE-based CNDC and PNC predicted by ML modeling with multi-source data fusion. Implications The active canopy sensor- and ML-based in-season N diagnosis and management strategy is more practical for applications under diverse on-farm conditions and has the potential to improve rice yield and ecological and economic benefits.
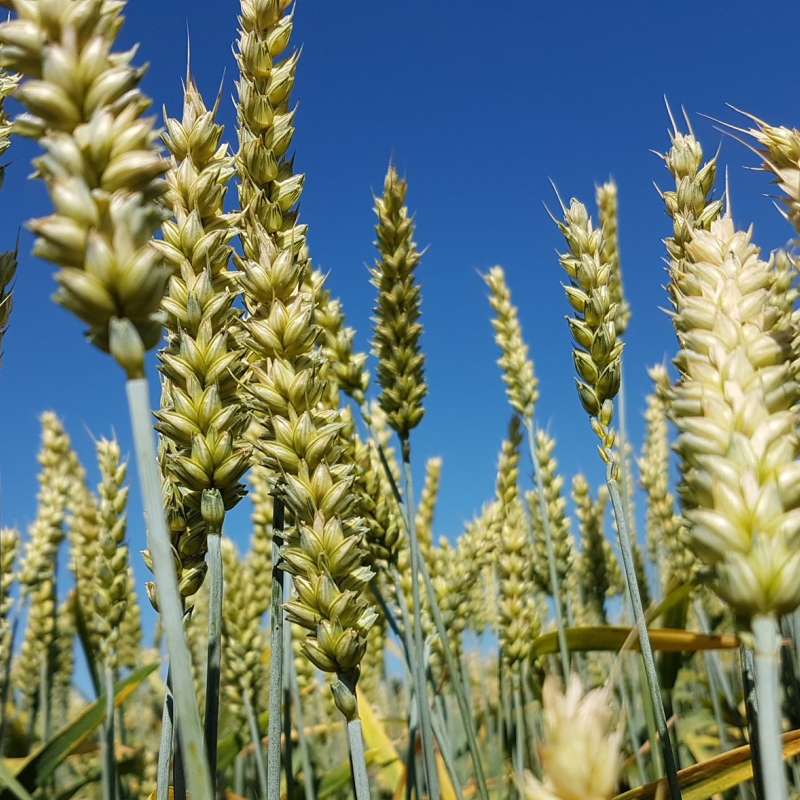
Division of Food Production and Society
Climate resilient and market adapted Norwegian winter wheat production
Interest in winter wheat is growing in Norway. Climate change is expected to expand the wheat producing regions, yet warmer, wetter conditions in autumn and winter will increase soil erosion and nutrient loss risks.
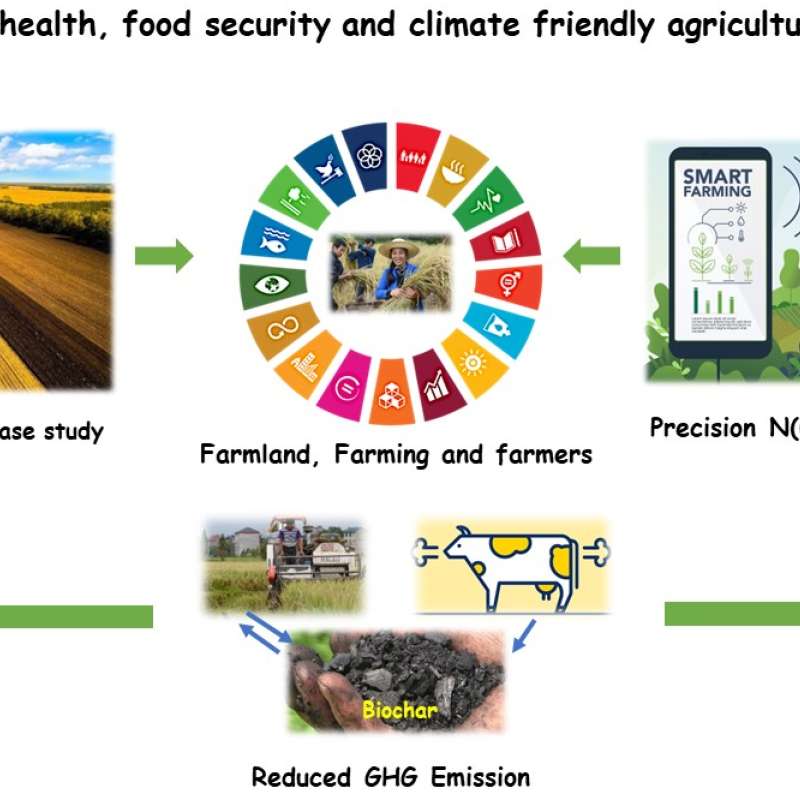
Division of Environment and Natural Resources
Sinograin III: Smart agricultural technology and waste-made biochar for food security, reduction of greenhouse gas (GHG) emission, and bio-and circular economy
The Sinograin III project’s overall objective is to contribute to the UN SDGs by widely implementing precision agriculture technologies and application of “waste-to-value” biochar products to achieve sustainable food production with minimized GHG emission, improve soil fertility and promote green growth/zero waste in modern agriculture in China.