Rasmus Astrup
Head of Research
Abstract
No abstract has been registered
Abstract
Intensification of forest management is seen as one important measure to increase carbon sequestration and contribute to balance CO2 emissions and mitigate climate change. Potential measures for forest management intensification include increasing the percentage of the area that is actively reforested after felling, planting at higher densities and with genetically improved material, nitrogen fertilization, and pre-commercial thinning. Here we assessed the mitigation potential of these practices in Norwegian forests from 2018 until the end of the 21st century. As a result, when these management practices were intensified, separately and simultaneously, carbon sequestration over the 80-year simulation period was larger than under current practices. Pre-commercial thinning gave the largest additional 80-year carbon dioxide removal increment and fertilization the smallest. The largest accumulated carbon dioxide removal potential occurred when intensifying all the proposed measures in one scenario and was estimated to be around 329.9 Tg CO2 by the end of the century, corresponding to offset more than six times Norway's total GHG emissions in 2022. If the intensification of these practices is considered separately, our results suggested that pre-commercial thinning and active reforestation after felling, in that order, should be prioritized as climate change mitigation measures, followed by genetic improvement, planting density and fertilization.
Abstract
RoadSens is a platform designed to expedite the digitalization process of forest roads, a cornerstone of efficient forest operations and management. We incorporate stereo-vision spatial mapping and deep-learning image segmentation to extract, measure, and analyze various geometric features of the roads. The features are precisely georeferenced by fusing post-processing results of an integrated global navigation satellite system (GNSS) module and odometric localization data obtained from the stereo camera. The first version of RoadSens, RSv1, provides measurements of longitudinal slope, horizontal/vertical radius of curvature and various cross-sectional parameters, e.g., visible road width, centerline/midpoint positions, left and right sidefall slopes, and the depth and distance of visible ditches from the road’s edges. The potential of RSv1 is demonstrated and validated through its application to two road segments in southern Norway. The results highlight a promising performance. The trained image segmentation model detects the road surface with the precision and recall values of 96.8 and 81.9 , respectively. The measurements of visible road width indicate sub-decimeter level inter-consistency and 0.38 m median accuracy. The cross-section profiles over the road surface show 0.87 correlation and 9.8 cm root mean squared error (RMSE) against ground truth. The RSv1’s georeferenced road midpoints exhibit an overall accuracy of 21.6 cm in horizontal direction. The GNSS height measurements, which are used to derive longitudinal slope and vertical curvature exhibit an average error of 5.7 cm compared to ground truth. The study also identifies and discusses the limitations and issues of RSv1, which provide useful insights into the challenges in future versions.
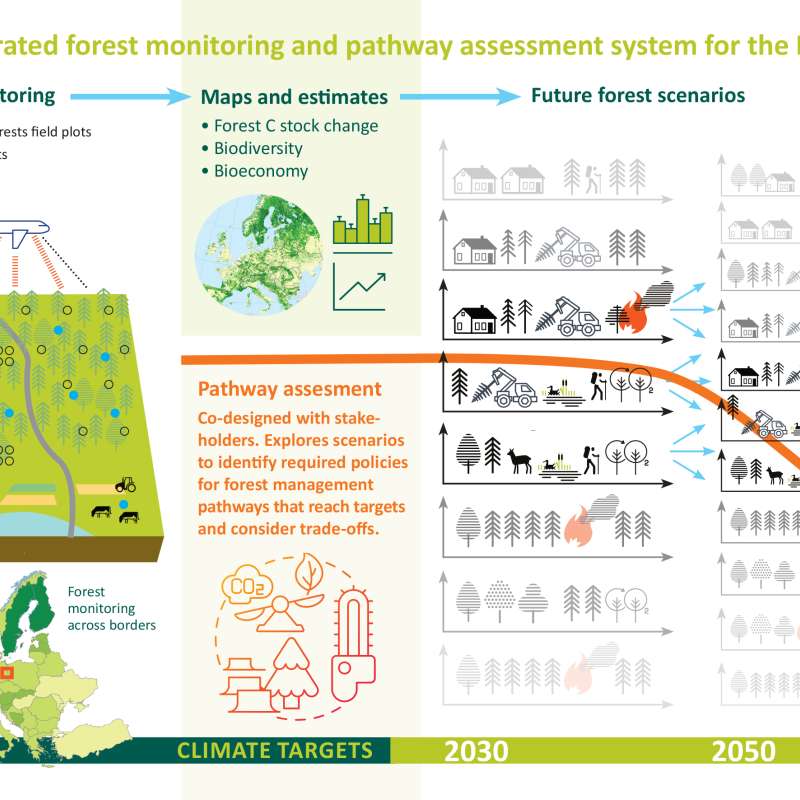
Division of Forest and Forest Resources
PathFinder
Towards an Integrated Consistent European LULUCF Monitoring and Policy Pathway Assessment Framework
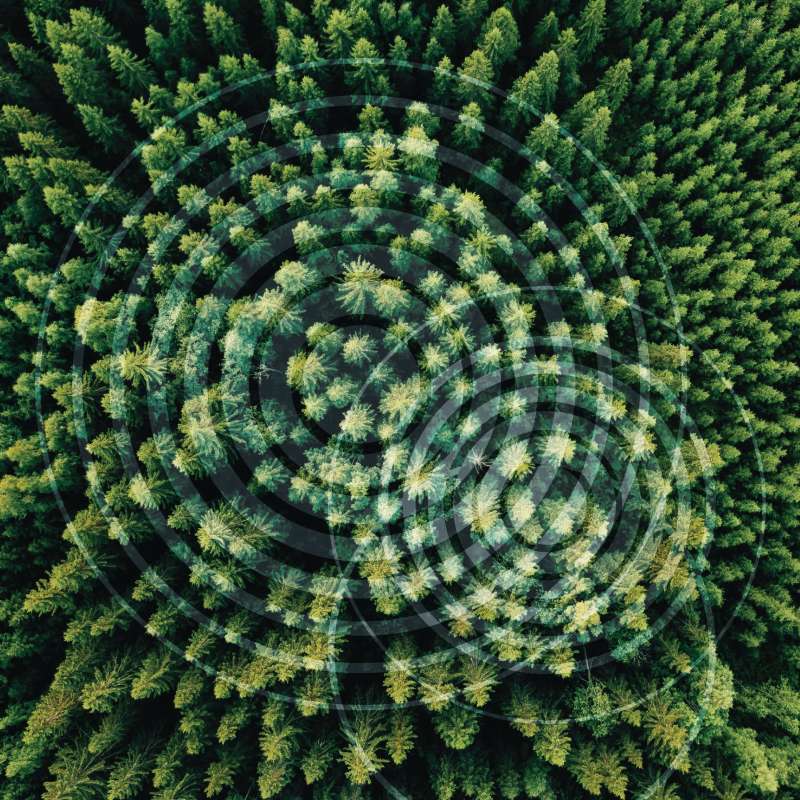
Division of Forest and Forest Resources
SFI SmartForest: Bringing Industry 4.0 to the Norwegian forest sector
SmartForest will position the Norwegian forest sector at the forefront of digitalization resulting in large efficiency gains in the forest sector, increased production, reduced environmental impacts, and significant climate benefits. SmartForest will result in a series of innovations and be the catalyst for an internationally competitive forest-tech sector in Norway. The fundamental components for achieving this are in place; a unified and committed forest sector, a leading R&D environment, and a series of progressive data and technology companies.